Method for predicting converter terminal point using artificial nurve network technology
An artificial neural network and technology prediction technology, which is applied in the field of predicting the end point of small and medium-sized converters, can solve the problems of many human factors, the inability to accurately and objectively reflect the actual situation of the end point of converter smelting, and the low hit rate of the end point.
- Summary
- Abstract
- Description
- Claims
- Application Information
AI Technical Summary
Problems solved by technology
Method used
Image
Examples
Embodiment Construction
[0015] figure 1 A schematic diagram of a three-layer BP network designed for programming, taking it as an example to combine figure 2 Let’s first describe its learning process:
[0016] Let the connection weight between node ai in LA layer and node bj in LB layer be Wij, the connection weight between node bj in LB layer and node ct in LC layer be Vjt, θj is the threshold of LB layer nodes, and γt is the threshold of LC layer nodes
[0017] (1) to W ij , θ j , V jt , gamma t Randomly assign a smaller value.
[0018] (2) For each pattern pair (A (K) , C (K) )(k=1, . . . , u), perform the following operations.
[0019] ① put A (K) the value of a i (K) ) into the LA layer node, according to the LA layer node activation value a i , a forward calculation:
[0020] b j = f ( Σ i = 1 m ...
PUM
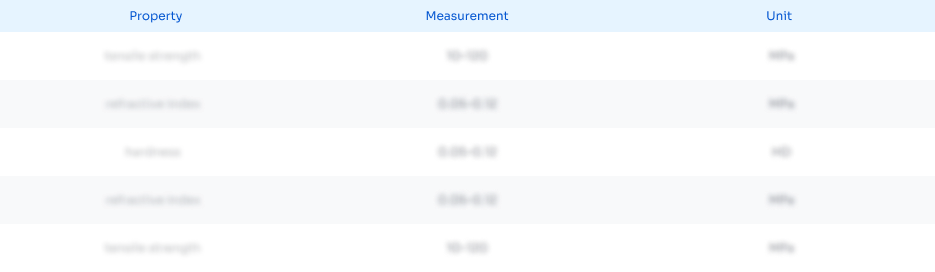
Abstract
Description
Claims
Application Information
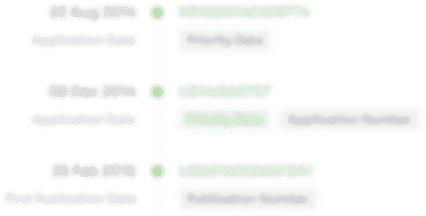
- R&D Engineer
- R&D Manager
- IP Professional
- Industry Leading Data Capabilities
- Powerful AI technology
- Patent DNA Extraction
Browse by: Latest US Patents, China's latest patents, Technical Efficacy Thesaurus, Application Domain, Technology Topic.
© 2024 PatSnap. All rights reserved.Legal|Privacy policy|Modern Slavery Act Transparency Statement|Sitemap