Quality of Medical Images Using Multi-Contrast and Deep Learning
a multi-contrast, deep learning technology, applied in the field of medical imaging, can solve the problems of not fully knowing how to make sure the model, the parameter tuning of different images is dependent, and the model is not fully trained, so as to shorten the imaging time and improve the quality of images
- Summary
- Abstract
- Description
- Claims
- Application Information
AI Technical Summary
Benefits of technology
Problems solved by technology
Method used
Image
Examples
Embodiment Construction
[0020]The current invention provides a method to improve the image quality of medical images. The invention provides a new end-to-end deep learning framework of taking raw image data and nonlinear filter results, which include denoised raw images with different denoising levels, and adding multi-contrast image data that have similar anatomy information with different contrasts, and generating improved image data with better quality in terms of resolution and SNR. The end-to-end framework of the current invention achieves better performance and faster speed.
[0021]In one embodiment, the invention improves the image quality of MRI imaging that typically has low SNR and resolution, for example arterial spin labeling (ASL) MRI images. The invention improves the image quality by using multi-contrast information from other images with the same anatomical structure but different contrasts, and using deep learning technique as an effective and efficient approach.
[0022]FIG. 1 shows a flow dia...
PUM
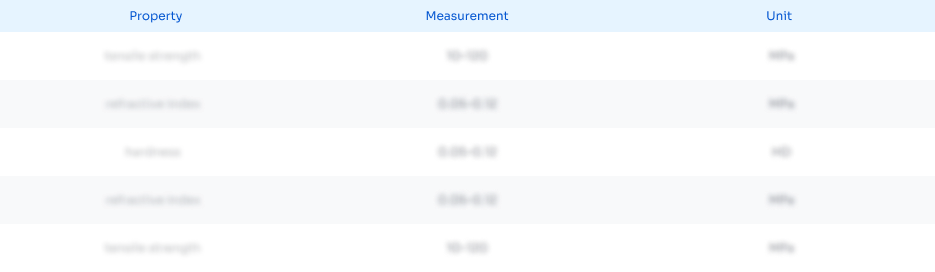
Abstract
Description
Claims
Application Information
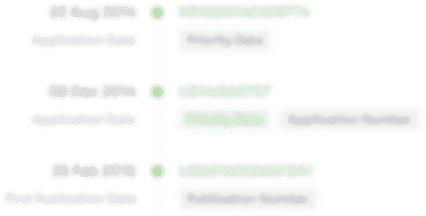
- R&D Engineer
- R&D Manager
- IP Professional
- Industry Leading Data Capabilities
- Powerful AI technology
- Patent DNA Extraction
Browse by: Latest US Patents, China's latest patents, Technical Efficacy Thesaurus, Application Domain, Technology Topic, Popular Technical Reports.
© 2024 PatSnap. All rights reserved.Legal|Privacy policy|Modern Slavery Act Transparency Statement|Sitemap|About US| Contact US: help@patsnap.com