Groove type reactor non-linear predication control method based on multi-kernel support vector machine
A nuclear support vector machine, support vector machine technology, applied in the direction of adaptive control, general control system, control/regulation system, etc., can solve the problems of modeling accuracy dependence, poor real-time performance, large amount of calculation, etc., to avoid online iteration The effect of approximate optimization, clear physical meaning, and simple identification process
- Summary
- Abstract
- Description
- Claims
- Application Information
AI Technical Summary
Problems solved by technology
Method used
Image
Examples
Embodiment Construction
[0030] The purpose and effects of the present invention will become more apparent by referring to the accompanying drawings in detail of the present invention.
[0031] 1. Nonlinear prediction model based on multi-core support vector machine
[0032] A support vector machine is a novel learning machine. Figure 2 shows the structural block diagram of the support vector machine itself. The basic idea of the support vector machine is to map the linearly inseparable low-dimensional space data into a linearly separable high-dimensional space through the nonlinear inner product kernel function, and perform linear regression fitting in this high-dimensional space.
[0033] The nonlinear model structure based on multi-kernel SVM is shown in Figure 3, which includes the concatenation of the dynamic part based on the linear kernel SVM and the static part based on the spline kernel SVM.
[0034] The linear dynamic part is described by
[0035] x(k+j|k)=f[x(k),...,x(k-n x +1), Δu(k+...
PUM
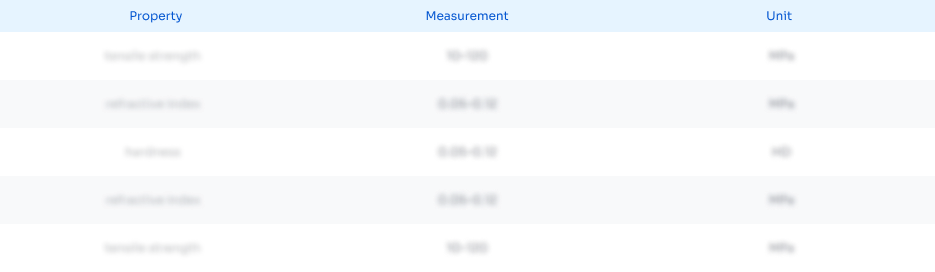
Abstract
Description
Claims
Application Information
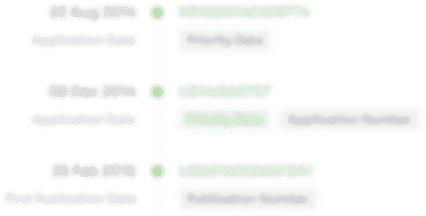
- R&D
- Intellectual Property
- Life Sciences
- Materials
- Tech Scout
- Unparalleled Data Quality
- Higher Quality Content
- 60% Fewer Hallucinations
Browse by: Latest US Patents, China's latest patents, Technical Efficacy Thesaurus, Application Domain, Technology Topic, Popular Technical Reports.
© 2025 PatSnap. All rights reserved.Legal|Privacy policy|Modern Slavery Act Transparency Statement|Sitemap|About US| Contact US: help@patsnap.com