kNN (k-nearest neighbor) fault detection method for online upgrading master sample model
A fault detection and master sample technology, applied in software testing/debugging, program loading/starting, program control devices, etc., can solve problems such as reducing modeling space, increasing data storage burden, and not being able to obtain the most accurate results, etc., to achieve Optimize data structure, improve data utilization, and reduce the effect of training sample sets
- Summary
- Abstract
- Description
- Claims
- Application Information
AI Technical Summary
Problems solved by technology
Method used
Image
Examples
Embodiment Construction
[0018] The present invention will be described in detail below in conjunction with examples.
[0019] In the present invention, in a large amount of normal historical data, the main sample is extracted by analyzing statistical characteristics such as covariance, correlation coefficient, and sample variance among original data samples, so that the original data space is compressed, and the newly collected normal data is substituted into The main sample model enables the main sample space to be updated online. Then, the k-Nearest Neighbor (kNN) rule is used for batch process fault detection based on master sample modeling with online upgrade. This technology solves the problems of large data volume, strong repeatability, high noise interference, and low data utilization rate of batch process modeling samples. In order to accurately select the main sample with obvious characteristics, it is necessary to analyze and process the statistical characteristics of the original sample. ...
PUM
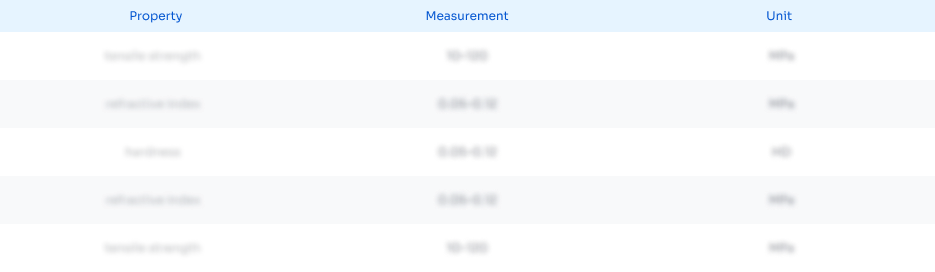
Abstract
Description
Claims
Application Information
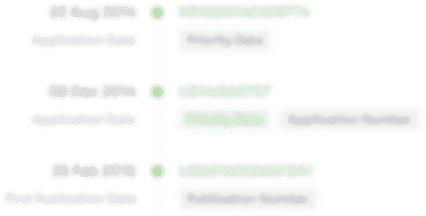
- R&D Engineer
- R&D Manager
- IP Professional
- Industry Leading Data Capabilities
- Powerful AI technology
- Patent DNA Extraction
Browse by: Latest US Patents, China's latest patents, Technical Efficacy Thesaurus, Application Domain, Technology Topic, Popular Technical Reports.
© 2024 PatSnap. All rights reserved.Legal|Privacy policy|Modern Slavery Act Transparency Statement|Sitemap|About US| Contact US: help@patsnap.com