Gear fault diagnosis method based on SVD decomposition and noise reduction and correlation EEMD entropy features
A fault diagnosis and correlation technology, applied in machine gear/transmission mechanism testing, special data processing applications, instruments, etc., can solve problems such as modal aliasing, difficulty in finding gear defect frequencies, and many iteration cycles
- Summary
- Abstract
- Description
- Claims
- Application Information
AI Technical Summary
Problems solved by technology
Method used
Image
Examples
Embodiment Construction
[0063] The present invention will be described in detail below in conjunction with the accompanying drawings and specific embodiments.
[0064] A gear fault diagnosis method based on SVD decomposition noise reduction and correlation EEMD entropy features, the process is as follows figure 1 As shown, the comprehensive application of correlation analysis, signal-to-noise ratio, SVD decomposition method, EEMD decomposition method, sample entropy theory and probability neural network theory,
[0065] Specifically, the following steps are included:
[0066] 1) Use the acceleration vibration sensor to collect the gear vibration signal of the test bench, and the obtained signal includes four fault types: normal gear, broken gear, few teeth, and gear wear;
[0067] 2) Using the SVD decomposition noise reduction method through correlation analysis and signal-to-noise ratio optimization to perform noise reduction processing on the four gear state signals of the simulated strong noise b...
PUM
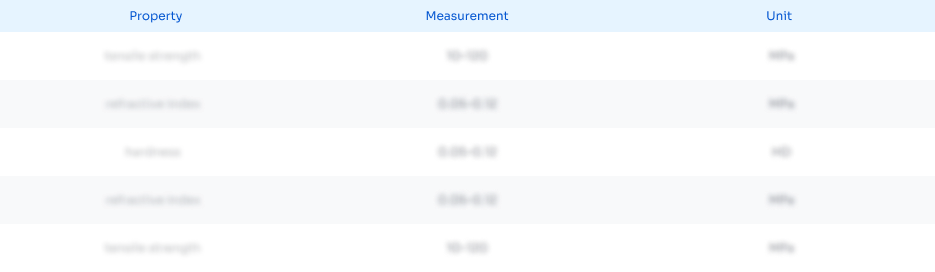
Abstract
Description
Claims
Application Information
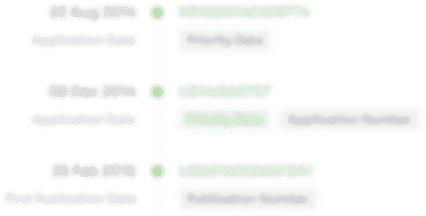
- R&D Engineer
- R&D Manager
- IP Professional
- Industry Leading Data Capabilities
- Powerful AI technology
- Patent DNA Extraction
Browse by: Latest US Patents, China's latest patents, Technical Efficacy Thesaurus, Application Domain, Technology Topic, Popular Technical Reports.
© 2024 PatSnap. All rights reserved.Legal|Privacy policy|Modern Slavery Act Transparency Statement|Sitemap|About US| Contact US: help@patsnap.com