Retinal vessel segmentation method of fundus image based on classification and regression tree and AdaBoost
A technology of retinal blood vessels and classification and regression trees, applied in image analysis, image enhancement, image data processing, etc., can solve the problems of uneven background fundus images, low accuracy, poor fundus image effects, etc.
- Summary
- Abstract
- Description
- Claims
- Application Information
AI Technical Summary
Problems solved by technology
Method used
Image
Examples
Embodiment 1
[0073] According to the method described in this article, the figure 2 Figure a is segmented, and the resulting manual marking and segmentation results are shown in Figure b and Figure c, respectively, and the obtained ROC curve is shown in Figure d; from figure 2 We can see the segmentation results, and the ROC curve of the method in this paper (the area between the curve and the X coordinate axis can evaluate the pros and cons of the segmentation algorithm, the larger the area, the better), from the area between the curve and the x axis AZ=0.9838 , it can be seen that the segmentation method in this paper is accurate and credible, and the accuracy reaches 0.9658, the sensitivity reaches 0.8358 and the specificity reaches 0.9820, which better proves that the segmentation method in this paper is accurate and credible.
Embodiment 2
[0075] According to the method described in this article, the image 3 Figure a is segmented, and the resulting manual marking and segmentation results are shown in Figure b and Figure c, respectively, and the obtained ROC curve is shown in Figure d; from image 3 We can see the segmentation results, and the ROC curve of the method in this paper (the area between the curve and the X coordinate axis can evaluate the pros and cons of the segmentation algorithm, the larger the area, the better), from the area between the curve and the x axis AZ=0.9802 , it can be seen that the segmentation method in this paper is accurate and credible, and the accuracy reaches 0.9711, the sensitivity reaches 0.7578 and the specificity reaches 0.9914, which better proves that the segmentation method in this paper is accurate and credible.
Embodiment 3
[0077] According to the method described in this article, the Figure 4 Figure a is segmented, and the resulting manual marking and segmentation results are shown in Figure b and Figure c, respectively, and the obtained ROC curve is shown in Figure d; from Figure 4 We can see the segmentation results, and the ROC curve of the method in this paper (the area between the curve and the X coordinate axis can evaluate the pros and cons of the segmentation algorithm, the larger the area, the better), from the area between the curve and the x axis AZ=0.9514 , it can be seen that the segmentation method in this paper is accurate and credible, and the accuracy reaches 0.9658, the sensitivity reaches 0.7011 and the specificity reaches 0.9747, which better proves that the segmentation method in this paper is accurate and credible.
[0078] Depend on Figure 2-Figure 4 The data shows that the area between the ROC curve and the x-axis is above 0.9500, the accuracy is above 0.9500, the spe...
PUM
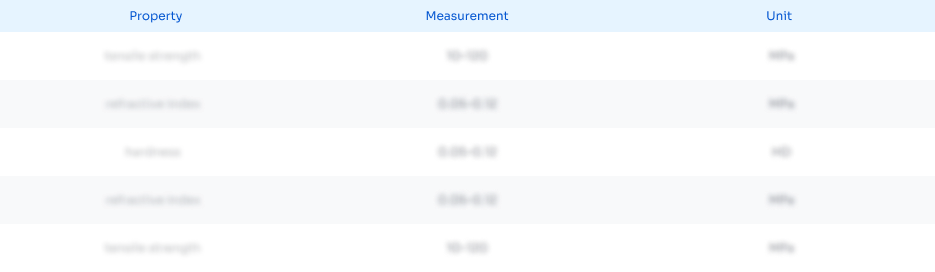
Abstract
Description
Claims
Application Information
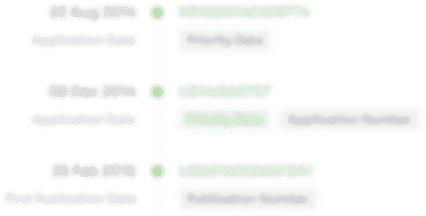
- R&D Engineer
- R&D Manager
- IP Professional
- Industry Leading Data Capabilities
- Powerful AI technology
- Patent DNA Extraction
Browse by: Latest US Patents, China's latest patents, Technical Efficacy Thesaurus, Application Domain, Technology Topic, Popular Technical Reports.
© 2024 PatSnap. All rights reserved.Legal|Privacy policy|Modern Slavery Act Transparency Statement|Sitemap|About US| Contact US: help@patsnap.com