Multi-dimensional time sequence classification method based on mahalanobis distance DTW
A time series and Mahalanobis distance technology, applied in the field of multi-dimensional time series classification based on Mahalanobis distance DTW, can solve the problems of unsatisfactory fixed point segmentation effect, inaccurate measurement results, and inaccurate classification results. The results are compact, the measurement results are not accurate enough, and the coupling degree is high.
- Summary
- Abstract
- Description
- Claims
- Application Information
AI Technical Summary
Problems solved by technology
Method used
Image
Examples
specific Embodiment approach 1
[0053] Specific Embodiment 1: A multidimensional time series classification method based on Mahalanobis distance DTW in this embodiment is specifically prepared according to the following steps:
[0054] Step 1: Segment the historical satellite telemetry data Y under the normal operating state of the satellite with the point of the argument mutation point as the mark, and obtain the normal multidimensional time series X={x 1 ,x 2 ,...,x j ,...x n}, where Y is n d row n a Columns of historical satellite telemetry data matrix, n d is the dimension value of multidimensional time series, n a is the number of data points of all historical satellite telemetry data, x j for n d row n len The column data matrix represents the jth sequence of X, j=1,2,...,n,n len is the length of the time series, n is the number of members in X;
[0055] Step 2. Multidimensional time series X={x obtained after segmentation 1 ,x 2 ,...,x j ,...x n}, through the hierarchical clustering meth...
specific Embodiment approach 2
[0067] Specific embodiment 2: The difference between this embodiment and specific embodiment 1 is that in step 1, the argument angle is one of the test parameters of the satellite telemetry data, and the change rule of the argument angle is from 0 ° to 360 °, which has an obvious cycle. , the argument angle value changes from 360° to 0° as the point of the argument angle mutation. Other steps and parameters are the same as those in Embodiment 1.
specific Embodiment approach 3
[0068] Specific embodiment three: the difference between this embodiment and specific embodiment one or two is that: in step one, the historical satellite telemetry data Y under the normal operation state of the satellite is segmented with the argument point of change, and the normal multi-dimensional time series is obtained X={x 1 ,x 2 ,...,x j ,...x n} The specific process is:
[0069] (1) When the argument angle reaches 360°, it will change to 0° and start to increase again, and the point from 360° to 0° is the abrupt change point of the argument angle;
[0070] (2) Record the corresponding time of the argument sudden change point;
[0071] (3) According to the time corresponding to the argument mutation point, extract the test data within the time corresponding to two adjacent argument mutation points as time series; where the multidimensional time series is composed of multiple time series; where the test data is partial Attitude angle, flywheel speed and bus voltage...
PUM
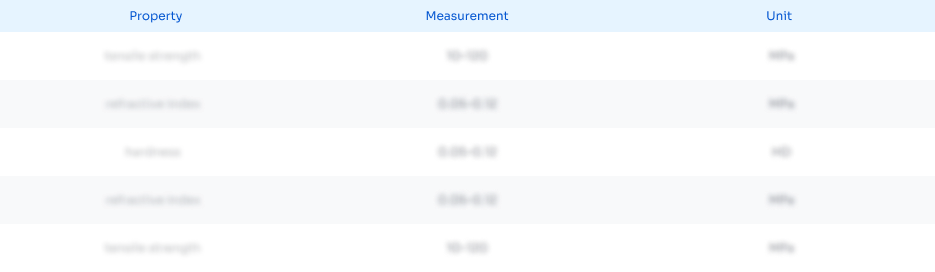
Abstract
Description
Claims
Application Information
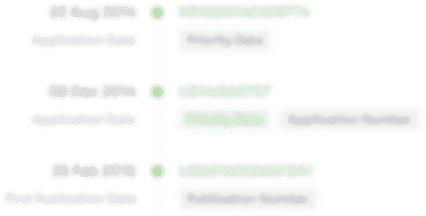
- R&D Engineer
- R&D Manager
- IP Professional
- Industry Leading Data Capabilities
- Powerful AI technology
- Patent DNA Extraction
Browse by: Latest US Patents, China's latest patents, Technical Efficacy Thesaurus, Application Domain, Technology Topic.
© 2024 PatSnap. All rights reserved.Legal|Privacy policy|Modern Slavery Act Transparency Statement|Sitemap