Deep learning cellular automaton model-based soil moisture content prediction method
A soil water content and cellular automaton technology, applied in the field of soil water content prediction, can solve problems such as difficulty in parallel scheduling and low data management efficiency, achieve broad industrial application prospects, improve prediction accuracy, and ensure the effect of robustness
- Summary
- Abstract
- Description
- Claims
- Application Information
AI Technical Summary
Problems solved by technology
Method used
Image
Examples
Embodiment Construction
[0051] The specific implementation manners of the present invention will be further described in detail below in conjunction with the accompanying drawings.
[0052] Such as figure 1 As shown, a kind of soil water content prediction method based on deep learning cellular automata model designed by the present invention, in practical application, due to various environmental variable data, training target soil area soil water content spatial distribution raster layer, soil The intercellular neighborhood action variables of water content correspond to the target soil area. Therefore, in the entire technical solution, the above-mentioned data are all in the form of raster layer data with the same size and resolution. And target soil area soil water content verification data, target soil area soil water content prediction data are sample point data, and each point corresponds to a specific position in the raster layer, and described prediction method comprises the following steps:...
PUM
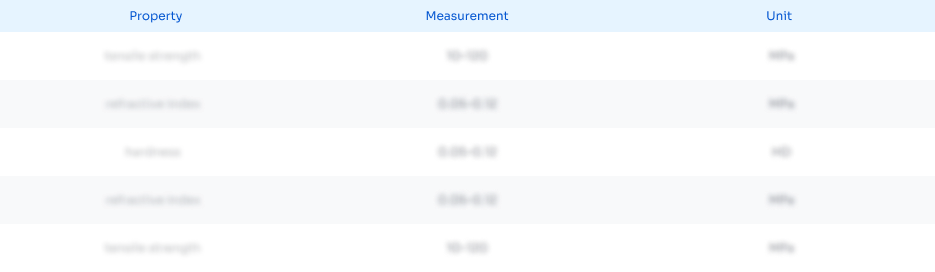
Abstract
Description
Claims
Application Information
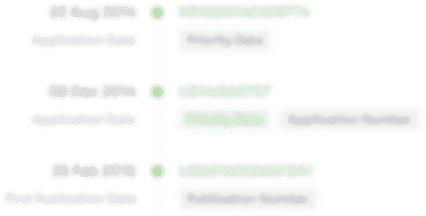
- R&D Engineer
- R&D Manager
- IP Professional
- Industry Leading Data Capabilities
- Powerful AI technology
- Patent DNA Extraction
Browse by: Latest US Patents, China's latest patents, Technical Efficacy Thesaurus, Application Domain, Technology Topic, Popular Technical Reports.
© 2024 PatSnap. All rights reserved.Legal|Privacy policy|Modern Slavery Act Transparency Statement|Sitemap|About US| Contact US: help@patsnap.com