Board thickness intelligent control method based on active learning
A technology of active learning and intelligent control, applied in non-electric variable control, self-adaptive control, material size control and other directions, can solve the problems of inability to improve the accuracy of the control system, poor system self-adaptation ability, and limited intelligent control level.
- Summary
- Abstract
- Description
- Claims
- Application Information
AI Technical Summary
Problems solved by technology
Method used
Image
Examples
Embodiment 1
[0088] Embodiment 1: training experiment of dynamic neural network
[0089] Such as figure 2 Construct a network model, set two neurons in the input layer of the network, three neurons in the hidden layer, and three neurons in the output layer, that is, the 2-3-3 structure, the learning step size is 0.03, the momentum factor is 0.2, and the activation function Use the S function. In the experiment, 5000 unlabeled samples were selected for training, and the number of initially randomly selected training samples was set to 200, and the active learning algorithm was used to select training samples that meet the requirements from 5000 marked samples and add them to the training sample set. The meta sensitivity threshold is , the maximum number of dynamic network training steps is , the output maximum error . Figure 4 Describes the dynamics of training through three methods: the active learning (MUSAL) algorithm based on the improved uncertainty sampling strategy, the...
Embodiment 2
[0090] Embodiment 2: the experiment of the intelligent control developmental model work based on active learning
[0091] Set the desired rigid thickness , requiring the maximum deviation allowed . The simulation curve of actual plate thickness output and plate thickness error changing with rolling time Such as Figure 5 and Figure 6 shown. The control method of the present invention can make the actual output thickness of the system reach the expected value , output at It starts to be stable when it is around , and can finally remain stable; the output error meets the requirements, at Within the range, the overshoot is very small; while the output of the passive learning dynamic network controller has finally reached a balance, but there is a slight error between the expected value, and it takes a long time to reach stability, and the overshoot is large. In this way, the high efficiency and applicability of the present invention are verified.
PUM
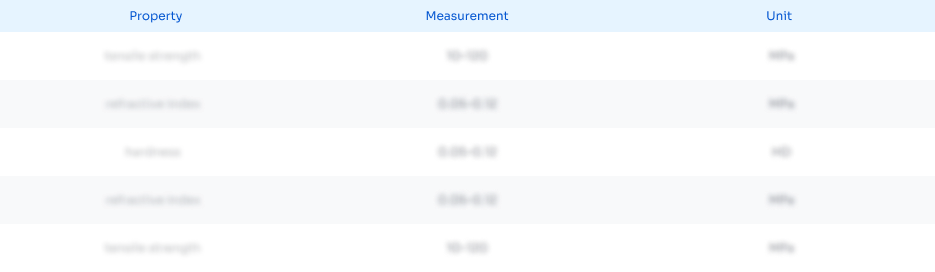
Abstract
Description
Claims
Application Information
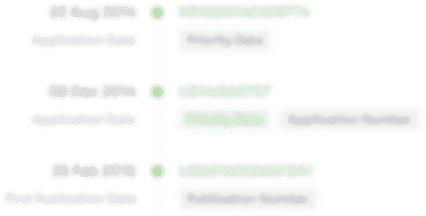
- R&D Engineer
- R&D Manager
- IP Professional
- Industry Leading Data Capabilities
- Powerful AI technology
- Patent DNA Extraction
Browse by: Latest US Patents, China's latest patents, Technical Efficacy Thesaurus, Application Domain, Technology Topic, Popular Technical Reports.
© 2024 PatSnap. All rights reserved.Legal|Privacy policy|Modern Slavery Act Transparency Statement|Sitemap|About US| Contact US: help@patsnap.com