A self-learning dynamic optimization method for batch processes driven by data differences
A dynamic optimization and data difference technology, applied in machine learning, program control, adaptive control, etc., can solve problems such as optimization performance limitations
- Summary
- Abstract
- Description
- Claims
- Application Information
AI Technical Summary
Problems solved by technology
Method used
Image
Examples
Embodiment Construction
[0047] This example takes a batch crystallization process as an example, and the method does not constitute a limitation of the scope of the present invention.
[0048] This method is divided into three parts. The first part is data collection and preprocessing. The second part is to calculate the initial optimization strategy. The third part is to calculate the recursive optimization strategy based on the updated batch data.
[0049]The block diagram of the implementation steps of this method is as follows: Image 6 As shown, the specific implementation steps and algorithms are as follows:
[0050] Step 1: For the complete batch crystallization process, select the temperature operating curve closely related to the product yield as the variable to be optimized, and collect 35 sets of temperature variable and final yield index data in batches. The data collection interval is 1 minute. figure 1 is an example of a temperature profile (partial) for a batch crystallization pro...
PUM
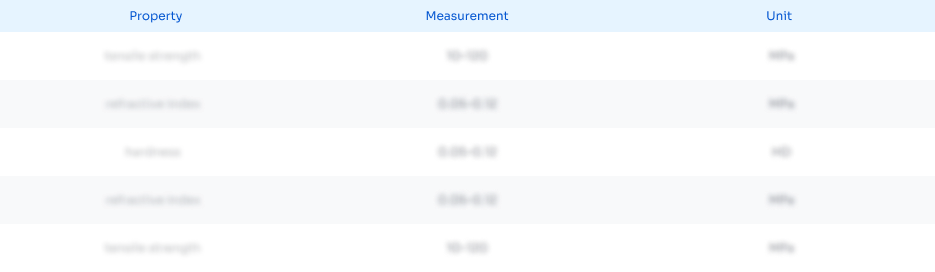
Abstract
Description
Claims
Application Information
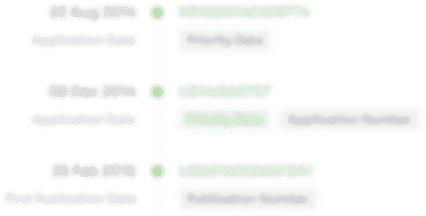
- R&D Engineer
- R&D Manager
- IP Professional
- Industry Leading Data Capabilities
- Powerful AI technology
- Patent DNA Extraction
Browse by: Latest US Patents, China's latest patents, Technical Efficacy Thesaurus, Application Domain, Technology Topic, Popular Technical Reports.
© 2024 PatSnap. All rights reserved.Legal|Privacy policy|Modern Slavery Act Transparency Statement|Sitemap|About US| Contact US: help@patsnap.com