Significant object detection method based on sparse subspace clustering and low-order expression
A low-rank representation and target detection technology, applied in the field of image processing, can solve problems such as difficult to detect salient targets, and achieve the effect of improving robustness
- Summary
- Abstract
- Description
- Claims
- Application Information
AI Technical Summary
Problems solved by technology
Method used
Image
Examples
Embodiment Construction
[0032] The embodiments and effects of the present invention will be further described in detail below in conjunction with the accompanying drawings.
[0033] Refer to attached figure 1 , the implementation steps of the present invention are as follows:
[0034] Step 1, input an image and perform superpixel segmentation.
[0035] (1a) Select an image from the MSRA-1000 database as the input image I;
[0036] (1b) Segment the input image I into N superpixels {p i |i=1,2,...,N}. Existing superpixel segmentation algorithm has SuperpixelLattice, Normalizedcuts, Turbopixels and SLIC etc., and wherein SLIC superpixel segmentation algorithm has obvious advantage on superpixel shape, boundary preservation and the computing speed of algorithm, the present invention selects SLIC superpixel segmentation algorithm for use Input image for segmentation.
[0037] Step 2, clustering the superpixels.
[0038]The Laplacian sparse subspace clustering algorithm adds the Laplacian item that c...
PUM
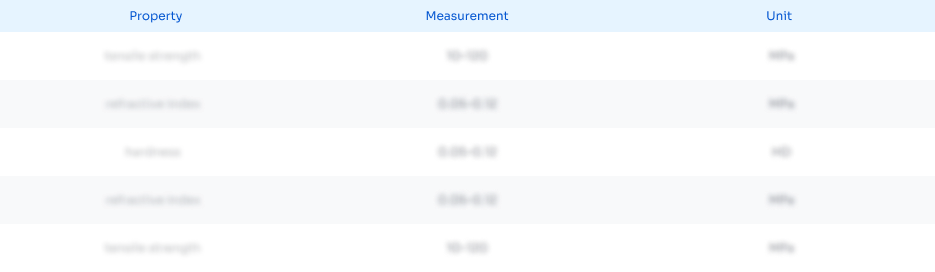
Abstract
Description
Claims
Application Information
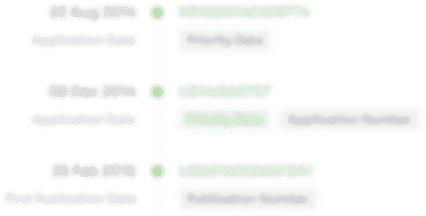
- R&D
- Intellectual Property
- Life Sciences
- Materials
- Tech Scout
- Unparalleled Data Quality
- Higher Quality Content
- 60% Fewer Hallucinations
Browse by: Latest US Patents, China's latest patents, Technical Efficacy Thesaurus, Application Domain, Technology Topic, Popular Technical Reports.
© 2025 PatSnap. All rights reserved.Legal|Privacy policy|Modern Slavery Act Transparency Statement|Sitemap|About US| Contact US: help@patsnap.com