LS-SVMs online learning method based on budget support vector set
A technology that supports vector sets and learning methods, applied in the fields of data mining and machine learning, can solve the problems of reducing model storage space and inability to effectively control model size, etc.
- Summary
- Abstract
- Description
- Claims
- Application Information
AI Technical Summary
Problems solved by technology
Method used
Image
Examples
Embodiment 1
[0053] Embodiment 1: Take the double helix classification problem as an example for illustration. Such as figure 1 Shown is a schematic diagram of a LS-SVMs online learning method based on a budget support vector set provided according to an embodiment of the present invention, and the online learning method contains the following steps:
[0054] Step 1: Determine the budget range using training samples. The specific steps are:
[0055] (1) Select the data set to be processed. In this embodiment, the reference data set Adult9 is taken as an example for illustration. Take the sample block size as 1, randomly select 2000 sample points from Adult9 to construct the training set and select 1000 sample points to construct the test set, and apply the standardized formula Preprocess the sample points. Choose Gaussian Radial Basis Functions As a kernel function, the kernel width parameter σ takes the default value, which is the dimension of the sample point.
[0056] (2) Determ...
Embodiment 2
[0079] Embodiment 2: Take the application of the sliding window technology as an example. The difference from Embodiment 1 is that in this embodiment, in step 3, the data stream is collected in the form of one-by-one, and the predictor is used to predict the samples in the data stream; in step 4, when collecting After getting the real label of the sample, compare the prediction output of the predictor, ignore the correct prediction sample, record the wrong prediction sample, add the wrong prediction sample to the support vector set, and remove the earliest support vector in the support vector set according to the time criterion to maintain The budget is stable.
[0080] Figures 5 to 8 In order to adopt the online learning method of the present invention and the existing stochastic budget perceptron method and the online gradient descent method to process the average online Schematic diagram of test accuracy comparison, by Figures 5 to 8 It can be seen that the test accura...
PUM
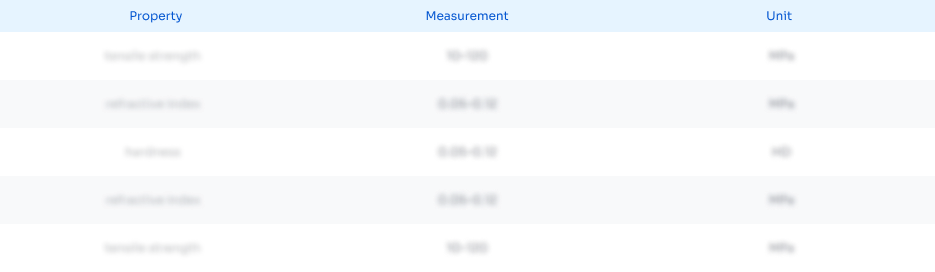
Abstract
Description
Claims
Application Information
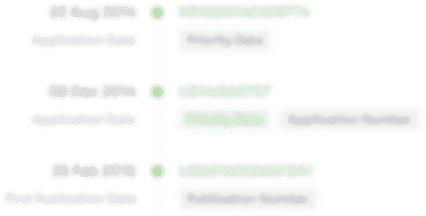
- R&D Engineer
- R&D Manager
- IP Professional
- Industry Leading Data Capabilities
- Powerful AI technology
- Patent DNA Extraction
Browse by: Latest US Patents, China's latest patents, Technical Efficacy Thesaurus, Application Domain, Technology Topic, Popular Technical Reports.
© 2024 PatSnap. All rights reserved.Legal|Privacy policy|Modern Slavery Act Transparency Statement|Sitemap|About US| Contact US: help@patsnap.com