Secondary protein structureprediction method based on deep neural network
A deep neural network and secondary structure technology, applied in the fields of feature learning, deep learning, neural network, protein structure prediction and sequence learning. It can improve the reliability of prediction, reduce the difficulty of parameter selection, and improve the robustness of the system.
- Summary
- Abstract
- Description
- Claims
- Application Information
AI Technical Summary
Problems solved by technology
Method used
Image
Examples
Embodiment 1
[0035] A protein secondary structure prediction method based on a deep neural network, comprising the following steps:
[0036] Step 1, the model training phase, which includes:
[0037] Obtain protein sequence combination features, position-specific scoring matrices (PSSM, position-specific scoring matrices), physical and chemical features as input, and train the self-encoder network to extract effective features;
[0038] Taking the protein sequence combination features of the independent training set as input and the corresponding secondary structure sequence as the target, the deep revertive neural network is trained by supervised learning to predict the secondary structure of each residue site.
[0039] Step 2, the prediction stage, which includes:
[0040] Input protein sequence features and predict the secondary structure of residues at each site.
[0041] In the above technical solution, the feature extraction in step 1 is from the encoder pre-training process, inclu...
Embodiment 2
[0064] see Figure 6 , a protein secondary structure prediction method based on a deep neural network, first, input a protein sequence feature combination sequence, which includes the combination features (PSSM, physicochemical features, etc.) of each residue site in the protein sequence. The input data needs to be preprocessed, which includes standardization, feature dimension alignment, etc. The final input is a protein sequence feature matrix.
[0065] The model training stage is to train the secondary structure prediction model. The specific process is as follows:
[0066] 1) Pre-trained self-encoding feature extraction network. A layer-by-layer training algorithm based on BP algorithm is used to train multiple autoencoders and stacked connections to form an autoencoder network. The network is a multi-layer deep neural network, such as figure 1 shown. The pre-trained self-encoder network is used for the preliminary feature extraction of the input protein combination f...
PUM
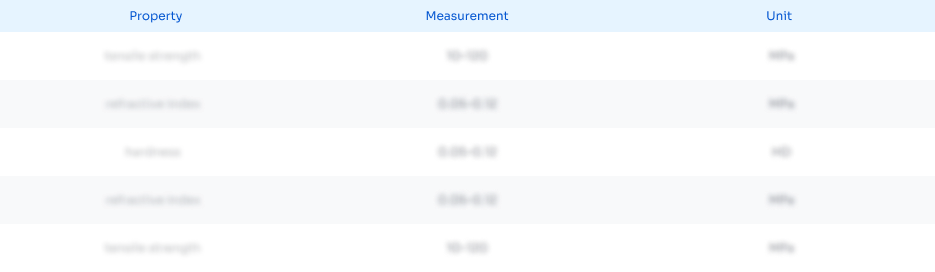
Abstract
Description
Claims
Application Information
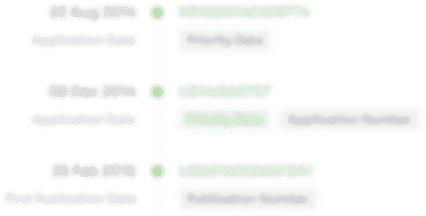
- R&D Engineer
- R&D Manager
- IP Professional
- Industry Leading Data Capabilities
- Powerful AI technology
- Patent DNA Extraction
Browse by: Latest US Patents, China's latest patents, Technical Efficacy Thesaurus, Application Domain, Technology Topic, Popular Technical Reports.
© 2024 PatSnap. All rights reserved.Legal|Privacy policy|Modern Slavery Act Transparency Statement|Sitemap|About US| Contact US: help@patsnap.com