Rolling bearing fault prediction method based on continuous deep belief network
A technology of deep belief networks and rolling bearings, which is applied in mechanical bearing testing, instruments, character and pattern recognition, etc. It can solve problems such as slow convergence speed, difficulty in effectively representing complex nonlinear functions, lack of theory in kernel function and parameter selection, etc.
- Summary
- Abstract
- Description
- Claims
- Application Information
AI Technical Summary
Problems solved by technology
Method used
Image
Examples
Embodiment 1
[0093] Produce a nonlinear, non-stationary time series x (t) by the chaotic Mackey-Glass differential delay equation, to verify the validity of the inventive method when analyzing and expressing nonlinear, non-stationary signals:
[0094] d x ( t ) d t = a x ( t - τ ) 1 + x 10 ( t - τ ) - b x ( t )
[0095] In this example, the initial conditions are set as a=0.25, b=0.1, x...
Embodiment 2
[0098] In this example, the rolling bearing life monitoring data in the prediction database of NASA is used to verify the accuracy of the method of the present invention in rolling bearing fault prediction. The experimental device is installed on a shaft with four bearings, driven by a DC motor, the speed is maintained at 2000rpm, and the radial load on the shaft is 6000 lbs. The bearing is a Rexnord ZA-115 double-row bearing, each bearing has 16 rolling elements, a diameter of 0.311 inches, a pitch diameter of 2.815 inches, and a contact angle of 15.17°. There are two PCB 353B33 high-sensitivity acceleration sensors placed vertically on each bearing to collect vibration acceleration signals. The vibration data is collected every 20 minutes by using the DAQCard-6062E acquisition card of NI Company. The sampling frequency of the data is 20kHz, and the data length of each sampling is 20480 points.
[0099] Figure 4 It is the whole life vibration signal of No. 3 rolling bearin...
PUM
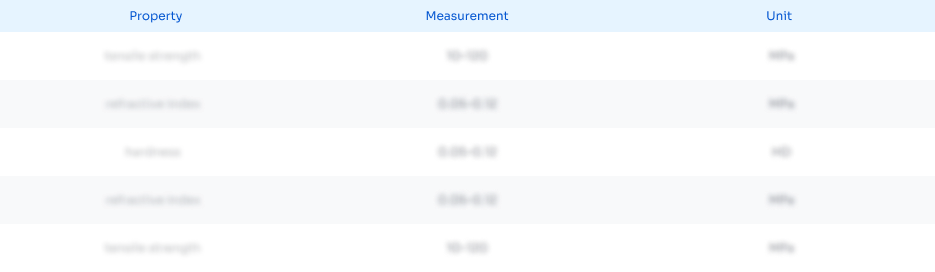
Abstract
Description
Claims
Application Information
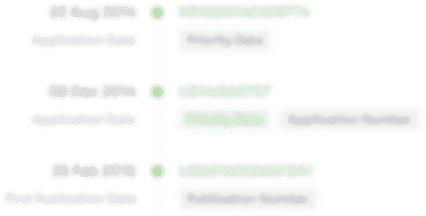
- R&D Engineer
- R&D Manager
- IP Professional
- Industry Leading Data Capabilities
- Powerful AI technology
- Patent DNA Extraction
Browse by: Latest US Patents, China's latest patents, Technical Efficacy Thesaurus, Application Domain, Technology Topic, Popular Technical Reports.
© 2024 PatSnap. All rights reserved.Legal|Privacy policy|Modern Slavery Act Transparency Statement|Sitemap|About US| Contact US: help@patsnap.com