Multi-modal feature selection and classification method based on hypergraph
A feature selection and classification method technology, applied in the field of neuroimaging and computer science, can solve the problems of only considering the paired relationship of samples, ignoring the high-order relationship of samples, etc., and achieve the effect of reducing information loss
- Summary
- Abstract
- Description
- Claims
- Application Information
AI Technical Summary
Problems solved by technology
Method used
Image
Examples
Embodiment
[0032] Such as figure 1 As shown, the specific implementation process includes five steps:
[0033] The first step is to construct a hypergraph. First, choose a sample in the brain image, and calculate the Euclidean distance between the sample and other samples; then sort the calculated Euclidean distances, and select the k samples with the closest Euclidean distance; The k samples of are connected into a hyperedge; for all other samples, similar processing is done. After the hypergraph is constructed, the corresponding correlation matrix, degree of vertices, degree of hyperedges and Laplacian matrix are calculated.
[0034] The second step is feature selection. Bring the calculated Laplacian matrix into the objective formula of (4) for optimization solution, and APG algorithm can be used for solution. The sparsity of the final weight matrix W is distributed in rows. The two columns w in the W matrix 1 ,w 2 Corresponding to the weight matrices of the two modes respectiv...
PUM
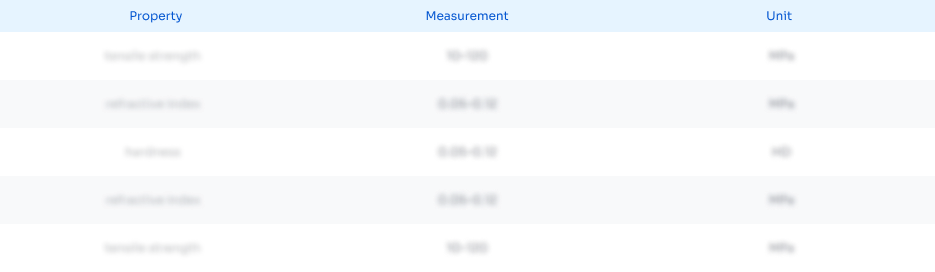
Abstract
Description
Claims
Application Information
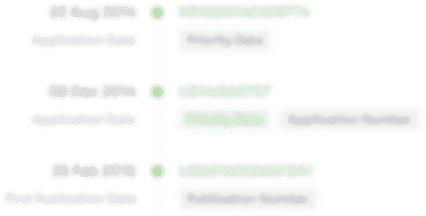
- R&D Engineer
- R&D Manager
- IP Professional
- Industry Leading Data Capabilities
- Powerful AI technology
- Patent DNA Extraction
Browse by: Latest US Patents, China's latest patents, Technical Efficacy Thesaurus, Application Domain, Technology Topic, Popular Technical Reports.
© 2024 PatSnap. All rights reserved.Legal|Privacy policy|Modern Slavery Act Transparency Statement|Sitemap|About US| Contact US: help@patsnap.com