Tool wear online monitoring method based on wavelet packet analysis and rbf neural network
A technology of neural network and tool wear, applied in manufacturing tools, measuring/indicating equipment, metal processing equipment, etc., can solve problems such as low resolution
- Summary
- Abstract
- Description
- Claims
- Application Information
AI Technical Summary
Problems solved by technology
Method used
Image
Examples
specific Embodiment approach
[0032] Reference attached Figure 1-6 Taking the milling process of general aviation material titanium alloy as an example, the specific implementation of the present invention will be described.
[0033] The present invention proposes a tool wear online monitoring method based on wavelet packet analysis and RBF neural network. Figure 1-2 Indicates the process and feature extraction process of the tool wear online monitoring method, which mainly includes 6 steps:
[0034] Step 1: Under a certain working condition, use constant cutting parameters to process the part. The tool mills the side of the titanium alloy workpiece with a radial distance of 1mm and an axial depth of 2mm for 58 times. The tool changes from initial wear to Blunt wear, measure the flank wear of the tool after each machining, and extract 50 sets of tool wear measurements as the output value of the neural network for training. At the same time, the Kistler 9123C rotary dynamometer is used to measure the cutting f...
PUM
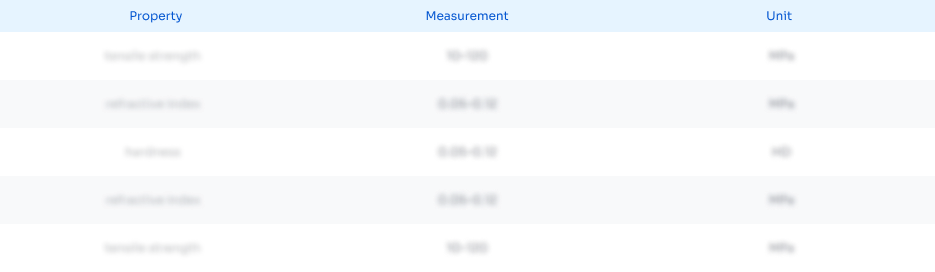
Abstract
Description
Claims
Application Information
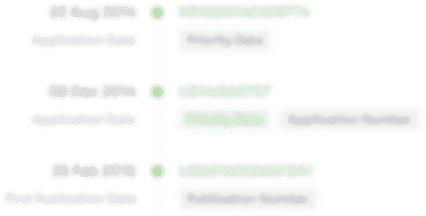
- R&D Engineer
- R&D Manager
- IP Professional
- Industry Leading Data Capabilities
- Powerful AI technology
- Patent DNA Extraction
Browse by: Latest US Patents, China's latest patents, Technical Efficacy Thesaurus, Application Domain, Technology Topic, Popular Technical Reports.
© 2024 PatSnap. All rights reserved.Legal|Privacy policy|Modern Slavery Act Transparency Statement|Sitemap|About US| Contact US: help@patsnap.com