Highly reflective surface defect detection method based on image processing and neural network classification
A neural network and image processing technology, applied in image data processing, biological neural network model, image analysis, etc., can solve problems such as high environmental requirements, high point cloud scanning accuracy and processing algorithm, and difficult data processing.
- Summary
- Abstract
- Description
- Claims
- Application Information
AI Technical Summary
Problems solved by technology
Method used
Image
Examples
Embodiment 1
[0068] 1. Data collection
[0069] After confirming the image acquisition equipment, lighting equipment and production space conditions used in the actual production environment, under this condition, image acquisition is performed on the tested surface with a certain number of inspected metal workpieces. During the acquisition process, the light source angle and angle are adjusted. Intensity, so that the required defect characteristics can be fully reflected in the collected images, and attention should be paid to the probability of qualified products and defective products in the tested parts used in data collection should be as close as possible to the qualified rate generated in the actual production process roughly consistent, and the defect characteristics and the location of the defect on the defective product should also cover all possible defects as much as possible.
[0070] 2. Front-end model debugging
[0071]Select some atlases of tested parts with typical defect...
PUM
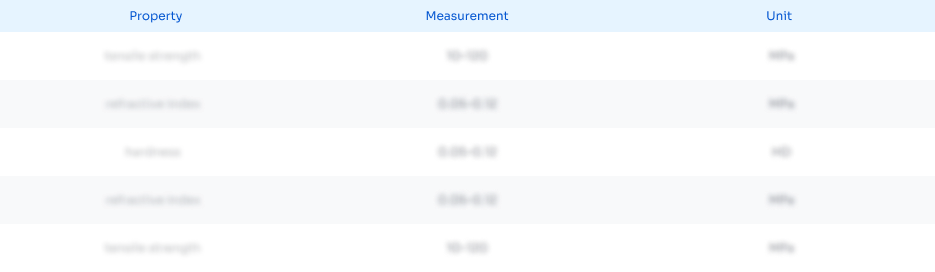
Abstract
Description
Claims
Application Information
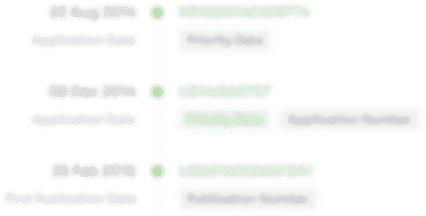
- R&D Engineer
- R&D Manager
- IP Professional
- Industry Leading Data Capabilities
- Powerful AI technology
- Patent DNA Extraction
Browse by: Latest US Patents, China's latest patents, Technical Efficacy Thesaurus, Application Domain, Technology Topic, Popular Technical Reports.
© 2024 PatSnap. All rights reserved.Legal|Privacy policy|Modern Slavery Act Transparency Statement|Sitemap|About US| Contact US: help@patsnap.com