Image denoising method and apparatus based on global and local prior cooperative constraints
An image and global technology, applied in the field of image denoising and device based on global and local prior joint constraints, can solve the problems of incomplete restoration of image fine structure and low image signal-to-noise ratio, etc., to remove image noise and improve image quality. The effect of the signal-to-noise ratio
- Summary
- Abstract
- Description
- Claims
- Application Information
AI Technical Summary
Problems solved by technology
Method used
Image
Examples
Embodiment 1
[0068] An image denoising method based on joint global and local prior constraints, which is used to denoise a single image Y to be processed, such as figure 1 shown, including the following steps:
[0069] Step 1, using formula I to establish a denoising model for the single image to be processed Y:
[0070]
[0071] Among them, X is the estimated pure image of the image Y to be processed, ||·|| w,* is the weighted kernel norm, Z represents the kernel Wiener filter image of image Y, λ WNN is the parameter of the set weighted kernel norm regularization term, λ KWF is the parameter of the kernel Wiener filter regularization term, λ WNN >0,λ KWF >0;
[0072] The observation model of the traditional image to be processed is usually expressed as:
[0073] Y=X+η Formula II
[0074] Among them, Y is the image to be processed, X is the clean image to be estimated, and η represents the noise.
[0075] In order to restore the pure image X to be estimated from the image Y to ...
Embodiment 2
[0134] This embodiment provides an image denoising device based on global and local prior joint constraints, which is used to denoise a single image Y to be processed, such as figure 2 As shown, the device includes:
[0135] The denoising model building module adopts the denoising model of formula I to remove noise from the image Y to be processed to obtain a denoising image
[0136]
[0137] Among them, X is the estimated pure image of the image Y to be processed, ||·|| w,* is the weighted kernel norm, Z represents the kernel Wiener filter image of image Y, λ WNN is the parameter of the set weighted kernel norm regularization term, λ KWF is the parameter of the kernel Wiener filter regularization term, λ WNN >0,λ KWF >0;
[0138] Decomposition module for rewriting Equation I into Equation III by using the scaled dual variable using the method of alternating direction multipliers:
[0139]
[0140] Among them, M is an auxiliary variable, ρ>0, and A is a Lagrangi...
Embodiment 3
[0159] In this embodiment, the commonly used pictures in the standard image test set are selected to test the method proposed in the present invention. Three-dimensional block matching (BM3D) algorithm and weighted kernel norm minimization denoising (WNNM) algorithm are the existing classic traditional denoising methods respectively. The deep convolutional neural network residual learning denoising (DnCNN) algorithm and the convolutional neural network-based fast and flexible image denoising (FFDNet) algorithm are currently the most advanced convolutional neural network-based denoising algorithms. The experimental results are shown in the table 1.
[0160] image 3 Pure image for Barbara, Figure 4-6 For the denoising method provided by the present invention when the noise is 25, 50, 75 image 3 Effect picture of denoising; Figure 7 For C-man pure image, Figure 8-10 For the denoising method provided by the present invention when the noise is 25, 50, 75 Figure 7 Image ...
PUM
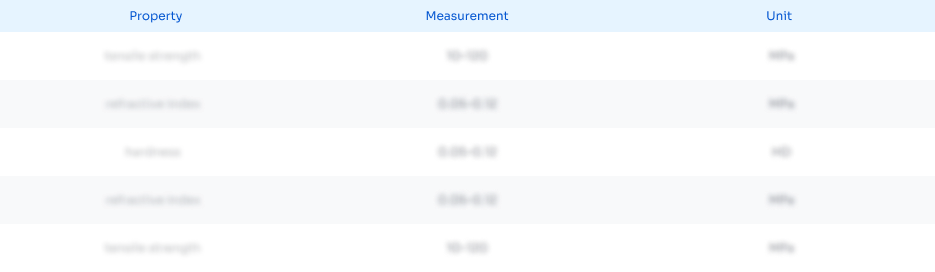
Abstract
Description
Claims
Application Information
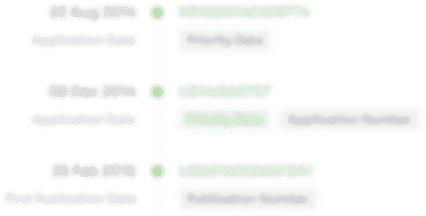
- R&D Engineer
- R&D Manager
- IP Professional
- Industry Leading Data Capabilities
- Powerful AI technology
- Patent DNA Extraction
Browse by: Latest US Patents, China's latest patents, Technical Efficacy Thesaurus, Application Domain, Technology Topic, Popular Technical Reports.
© 2024 PatSnap. All rights reserved.Legal|Privacy policy|Modern Slavery Act Transparency Statement|Sitemap|About US| Contact US: help@patsnap.com