Modulation recognition method for extracting time-frequency image features by joint entropy and pre-training CNN
A technology of time-frequency image and modulation recognition, which is applied to pattern recognition in signals, character and pattern recognition, instruments, etc. It can solve the problem of low training accuracy, achieve high recognition rate, improve system stability, and avoid sudden drop in quantity Effect
- Summary
- Abstract
- Description
- Claims
- Application Information
AI Technical Summary
Problems solved by technology
Method used
Image
Examples
Embodiment Construction
[0054] The present invention will be further described below in conjunction with accompanying drawing:
[0055] First, the Choi-Williams distribution (CWD) time-frequency transformation is performed on the 9 types of radar signal sets to be identified to obtain a time-frequency image; then based on the pre-trained convolutional neural network model imagenet-vgg-verydeep-19 provided by MatConvNet official website, the time-frequency The image size is adjusted to 224×224×3, so that the parameters of the pre-trained network model remain unchanged, and the FT-VGGNet-fc6 feature migration extraction module is composed of its Input input layer to the fc6 fully connected layer; then the adjusted image is sent to the feature The migration extraction module outputs the time-frequency image features of the radar signal, and applies PCA to reduce the feature dimension to 95 features; then grayscales the adjusted image, manually extracts the Renyi entropy of the processed image, and combin...
PUM
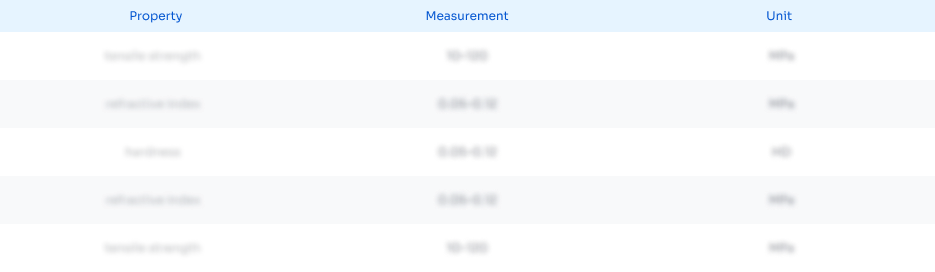
Abstract
Description
Claims
Application Information
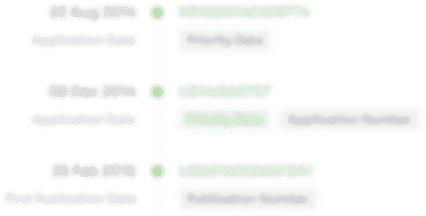
- R&D Engineer
- R&D Manager
- IP Professional
- Industry Leading Data Capabilities
- Powerful AI technology
- Patent DNA Extraction
Browse by: Latest US Patents, China's latest patents, Technical Efficacy Thesaurus, Application Domain, Technology Topic, Popular Technical Reports.
© 2024 PatSnap. All rights reserved.Legal|Privacy policy|Modern Slavery Act Transparency Statement|Sitemap|About US| Contact US: help@patsnap.com