A variable feature extraction method for NOx prediction model of thermal power plant based on contrast method
A predictive model and feature extraction technology, applied in neural learning methods, biological neural network models, complex mathematical operations, etc., can solve problems such as complex formation mechanism and inconspicuous extraction effect, achieve wide application range, reduce calculation time consumption, The effect of dimensionality reduction
- Summary
- Abstract
- Description
- Claims
- Application Information
AI Technical Summary
Problems solved by technology
Method used
Image
Examples
Embodiment 1
[0048] see Figure 1~4 , a kind of variable feature extraction method of thermal power plant NOx prediction model based on the comparison method, comprising the following steps:
[0049] S1) Collect the design data of the target boiler, analyze the mechanism of the combustion process of pulverized coal in combination with the actual operating environment, and find out the influence of NO X The main factors of emissions, determine the input variables of the model, and export a certain amount of historical data for denoising and filtering preprocessing. The determined input variables include: 1-5 layers of SOFA opening, ammonia escape, ammonia injection flow, ammonia flow valve opening, pipeline flow regulating valve setting value, regulating valve adjustment command, reactor inlet pressure, flue gas flow, furnace chamber Outlet flue gas temperature, total coal volume, total air volume, secondary air temperature at air preheater outlet of the unit, instantaneous flow rate of co...
Embodiment 2
[0064] This embodiment is a further elaboration on the basis of Embodiment 1, and S2 comprises the following steps:
[0065] First, the final evaluation function of the S-MIFS algorithm is obtained:
[0066]
[0067] In the above formula, f i ∈F is the candidate variable, c is the leading variable, S j ∈S is the selected variable. β is the penalty factor, and |S| is the norm of S or the number of selected variables of the selected set S.
[0068] S2-1, initialization setting, F is the variable set to be selected, S is the selected variable set (blank), and c is the leading variable;
[0069] S2-2. Calculate f i Mutual information with the leading variable c, take out the variable f corresponding to the maximum value i Stored in S; its mathematical expression is as follows:
[0070]
[0071] where p(f i ) for f i The marginal probability distribution of c, p(c) is the marginal probability distribution of c, p(f i , c) is f i and the joint probability distributio...
Embodiment 3
[0077] This embodiment is a further elaboration on the basis of Embodiment 1, and S3 comprises the following steps:
[0078] S3-1. Arranging the original data in rows to form a matrix X;
[0079]
[0080] S3-2. Perform data standardization on X to make its mean value zero;
[0081] S3-3. Find the covariance matrix C of X;
[0082] S3-4. Arrange the eigenvectors according to the eigenvalues from large to small, and take the first k to form a matrix P by row;
[0083] S3-5. By calculating Y=PX, the dimensionally reduced data Y is obtained;
[0084] S3-6. Calculate the contribution rate of each characteristic root.
[0085] Obtain NO after screening through the calculation of Example 1-Example 3 X The root mean square error between the actual concentration value and the test value is 3.3204, which is slightly lower than the accuracy before screening, but the time is obviously reduced by about 30% than before. The method of the invention does have positive effects in the...
PUM
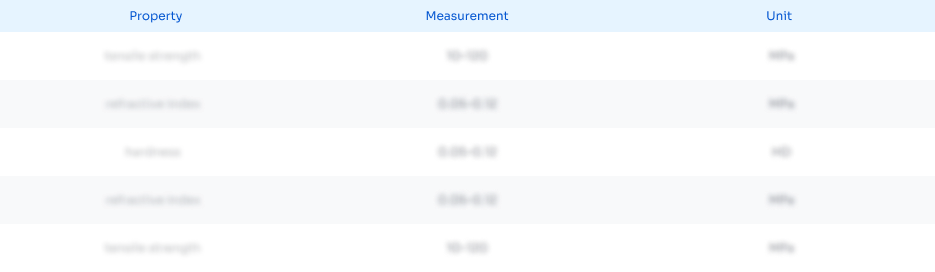
Abstract
Description
Claims
Application Information
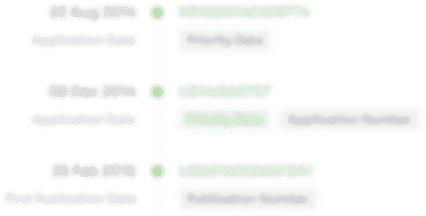
- Generate Ideas
- Intellectual Property
- Life Sciences
- Materials
- Tech Scout
- Unparalleled Data Quality
- Higher Quality Content
- 60% Fewer Hallucinations
Browse by: Latest US Patents, China's latest patents, Technical Efficacy Thesaurus, Application Domain, Technology Topic, Popular Technical Reports.
© 2025 PatSnap. All rights reserved.Legal|Privacy policy|Modern Slavery Act Transparency Statement|Sitemap|About US| Contact US: help@patsnap.com