An image de-fog method based on depth neural network
A deep neural network and image technology, applied in the field of image processing technology and deep learning, can solve the problem that pictures cannot achieve satisfactory dehazing effect, and achieve the effect of high dehazing efficiency, easy implementation and fast speed.
- Summary
- Abstract
- Description
- Claims
- Application Information
AI Technical Summary
Problems solved by technology
Method used
Image
Examples
Embodiment 1
[0047] In order to achieve high-quality image defogging, an embodiment of the present invention proposes an image defogging method based on a deep neural network, see figure 1 , see the description below:
[0048] 101: Select the global atmospheric light and atmospheric scattering coefficient, use the depth of field to generate the fog map and its transmittance map; make the fog-free map, fog map and transmittance map into a training set;
[0049] 102: Based on the encoder-decoder architecture, build a generator network including the estimated transmittance sub-network and the dehaze sub-network; and use the linearity of the confrontation loss function, the transmittance L1 norm loss function and the dehaze map L1 norm loss function combined training generator;
[0050] 103: Construct a discriminator network based on the convolutional layer, sigmoid activation function and LeakyReLU function; use the real fog-free image and the defogged image generated by the defogging sub-netw...
Embodiment 2
[0092] The scheme in Embodiment 1 is introduced in detail below in conjunction with specific drawings and calculation formulas, see the following description for details:
[0093] 201: Select the global atmospheric light and atmospheric scattering coefficient, use the depth of field to generate the fog map and its transmittance map; make the fog-free map, fog map and transmittance map into a training set;
[0094] 202: Construct a generator network based on the encoder-decoder architecture including the estimated transmittance subnetwork and the dehaze subnetwork; and use the linearity of the confrontation loss function, the transmittance L1 norm loss function and the dehaze map L1 norm loss function combined training generator;
[0095] 203: Construct a discriminator network based on the convolutional layer, sigmoid activation function, and LeakyReLU function; use the real haze-free image and the dehazed image generated by the dehazing sub-network as positive and negative sam...
Embodiment 3
[0117] The scheme in embodiment 1 and 2 is carried out feasibility verification by experimental data below, see the following description for details:
[0118] Select 3 real scenes with fog images and use the fog removal method of the present invention to remove fog, Figure 4 , Figure 5 and Figure 6 All are real scenes with fog and dehazed images. It can be seen from the results that this method has an ideal dehazing effect on the foggy image of the real scene.
PUM
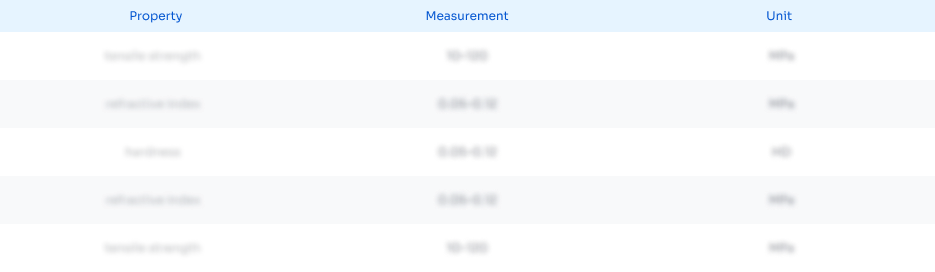
Abstract
Description
Claims
Application Information
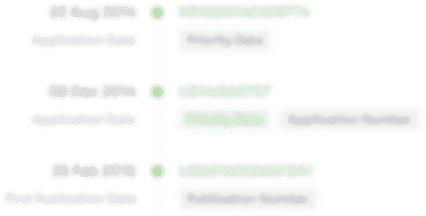
- R&D
- Intellectual Property
- Life Sciences
- Materials
- Tech Scout
- Unparalleled Data Quality
- Higher Quality Content
- 60% Fewer Hallucinations
Browse by: Latest US Patents, China's latest patents, Technical Efficacy Thesaurus, Application Domain, Technology Topic, Popular Technical Reports.
© 2025 PatSnap. All rights reserved.Legal|Privacy policy|Modern Slavery Act Transparency Statement|Sitemap|About US| Contact US: help@patsnap.com