A heart left ventricle segmentation method based on a deep full convolutional neural network
A convolutional neural network and left ventricle technology, applied in the field of heart left ventricle segmentation, can solve problems such as low segmentation accuracy and loss of fine object information, and achieve the effect of time-consuming and labor-intensive solutions
- Summary
- Abstract
- Description
- Claims
- Application Information
AI Technical Summary
Problems solved by technology
Method used
Image
Examples
Embodiment Construction
[0024] In order to describe the present invention more clearly, the technical solution of the present invention will be described in detail below in conjunction with the accompanying drawings and specific embodiments.
[0025] The present invention is based on the heart left ventricle segmentation method of deep fully convolutional neural network, and specific implementation steps are as follows:
[0026] S1. Obtain the complete cardiac magnetic resonance image of the subject, as well as the corresponding manually drawn left ventricular endocardial contour line. The subject's complete cardiac MRI short-axis images only contain ordinary cine sequences, such as figure 2 As shown, that is, the coronal, sagittal, and axial three-direction positioning maps are simultaneously made on the subject by the magnetic resonance instrument. The imaging range is from the bottom of the heart and the root of the great blood vessels to the apex of the heart. We only screen short-axis cardiac i...
PUM
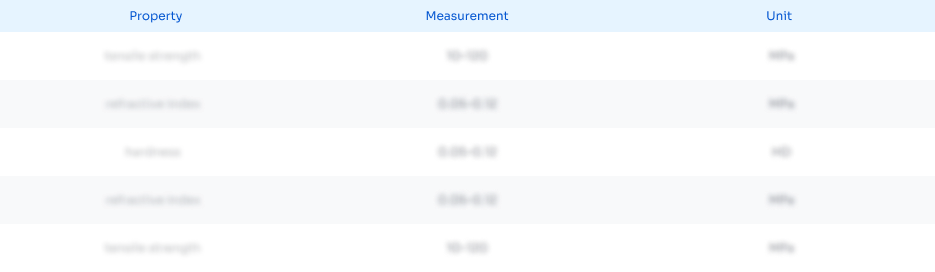
Abstract
Description
Claims
Application Information
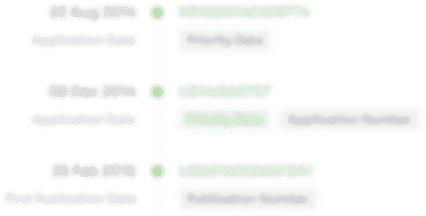
- R&D Engineer
- R&D Manager
- IP Professional
- Industry Leading Data Capabilities
- Powerful AI technology
- Patent DNA Extraction
Browse by: Latest US Patents, China's latest patents, Technical Efficacy Thesaurus, Application Domain, Technology Topic, Popular Technical Reports.
© 2024 PatSnap. All rights reserved.Legal|Privacy policy|Modern Slavery Act Transparency Statement|Sitemap|About US| Contact US: help@patsnap.com