Fault state recognition method for port machine wheel bearing
A fault state, wheel bearing technology, applied in the testing of mechanical components, testing of machine/structural components, measuring devices, etc., can solve problems such as lack of generalization, and achieve the effect of accurate and effective detection
- Summary
- Abstract
- Description
- Claims
- Application Information
AI Technical Summary
Problems solved by technology
Method used
Image
Examples
Embodiment approach
[0027] As an embodiment of the present invention, the time domain features include: mean value, root mean square value, peak value, variance, peak-to-peak value, signal energy, crest factor, kurtosis, pulse index, skewness and margin coefficient.
[0028] Frequency domain features include: power spectrum sum, power spectrum mean, power spectrum variance, power spectrum skewness, power spectrum kurtosis, and power spectrum peak.
[0029] Time-frequency domain features include: time-frequency total energy, time-frequency energy distribution variance over time, time-frequency energy distribution skewness over time, time-frequency energy distribution kurtosis over time, time-frequency energy distribution variance over frequency, time-frequency energy over frequency Distribution skewness, time-frequency energy distribution kurtosis with frequency.
[0030] Step 4: Using the combination method of Pearson correlation coefficient and residual analysis, carry out correlation analysis o...
PUM
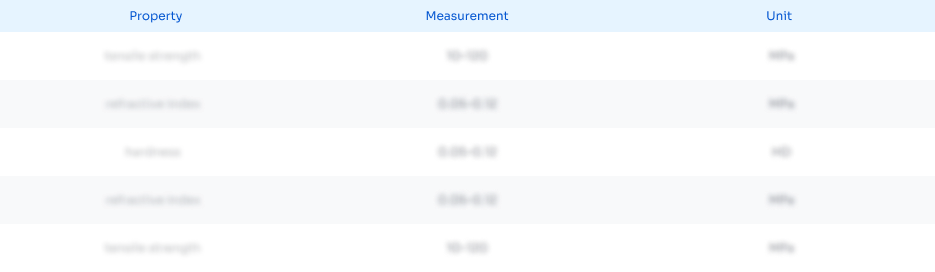
Abstract
Description
Claims
Application Information
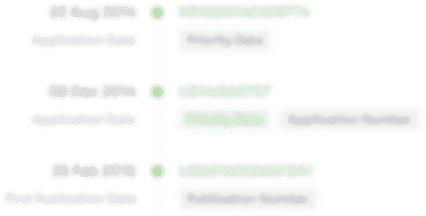
- R&D Engineer
- R&D Manager
- IP Professional
- Industry Leading Data Capabilities
- Powerful AI technology
- Patent DNA Extraction
Browse by: Latest US Patents, China's latest patents, Technical Efficacy Thesaurus, Application Domain, Technology Topic, Popular Technical Reports.
© 2024 PatSnap. All rights reserved.Legal|Privacy policy|Modern Slavery Act Transparency Statement|Sitemap|About US| Contact US: help@patsnap.com