A multi-task collaborative identification method and system
A collaborative identification, multitasking technology, applied in multimedia data indexing, multimedia data retrieval, special data processing applications, etc., can solve the real identification task of a huge number of parameters, difficult to achieve rapid and balanced configuration of network resources, continuous data flow input Poor performance, etc.
- Summary
- Abstract
- Description
- Claims
- Application Information
AI Technical Summary
Problems solved by technology
Method used
Image
Examples
Embodiment 1
[0081] like figure 1As shown, a multi-task collaborative identification system disclosed in Embodiment 1 of the present invention includes:
[0082] The general feature extraction module is used to establish a time synchronization matching mechanism for multi-source heterogeneous data, realize a multi-source data association description model based on potential high-level shared semantics, realize efficient support between different channel data, information complementarity, and maximize the realization of data de-redundancy;
[0083] The deep collaborative feature learning module is used to build a long-term dependent generative memory model, explore a semi-supervised continuous learning system based on collaborative attention and deep autonomy, realize dynamic self-learning with selective memory and forgetting ability, and achieve the goal of understanding existing learning models. The effect of incremental performance improvements;
[0084] The intelligent multi-task deep...
Embodiment 2
[0093] Embodiment 2 of the present invention provides a method for multi-task discrimination using the above system, the method includes: a general feature description of massive multi-source audiovisual media perception data, including establishing a time synchronization matching mechanism for multi-source heterogeneous data, and realizing Multi-source data association description model with potential high-level shared semantics; deep collaborative feature learning for long-term memory of continuously input streaming media data, including building long-term dependent generative memory models, and exploring semi-supervised continuous learning based on collaborative attention and deep autonomy system; intelligent multi-task deep collaborative enhanced feedback recognition model under the adaptive framework, including the theory of context-aware computing based on the cooperative work of agents, and the introduction of adaptive deep collaborative enhanced feedback and multi-task j...
Embodiment 3
[0100] like figure 1 As shown, the third embodiment of the present invention provides a multi-task collaborative identification method.
[0101] First, a general feature description method for multi-source audiovisual media perception data is established by using the migration algorithm.
[0102] In order to achieve efficient collaborative analysis for different visual and auditory tasks, a highly robust and versatile feature description is extracted from the multi-source visual and auditory perception data. As a prototype feature for subsequent collaborative learning, it is first necessary to analyze the visual and auditory perception data Features. Most of the actually acquired audio data is a one-dimensional time series, which is mainly descriptive in its spectral-temporal cues. It is necessary to use the spectral transformation of the auditory receptive domain combined with the prosody information of adjacent audio frames to describe. The visual perception data are mostl...
PUM
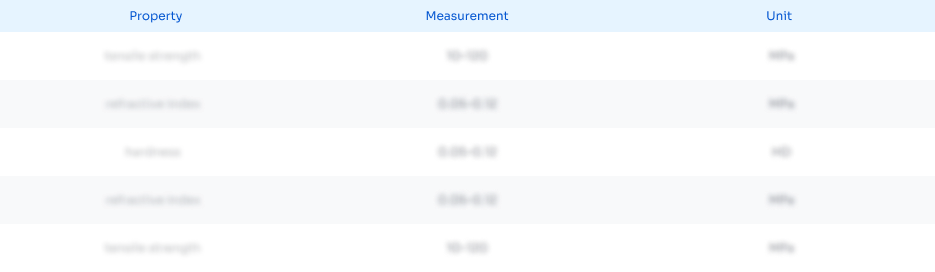
Abstract
Description
Claims
Application Information
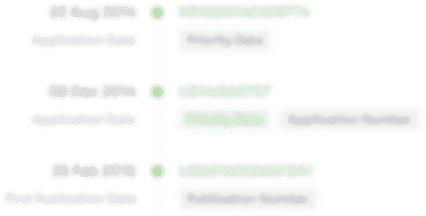
- R&D Engineer
- R&D Manager
- IP Professional
- Industry Leading Data Capabilities
- Powerful AI technology
- Patent DNA Extraction
Browse by: Latest US Patents, China's latest patents, Technical Efficacy Thesaurus, Application Domain, Technology Topic.
© 2024 PatSnap. All rights reserved.Legal|Privacy policy|Modern Slavery Act Transparency Statement|Sitemap