Pedestrian detection network, model training method, detection method, medium and equipment
A pedestrian detection and network model technology, applied in character and pattern recognition, instruments, computer parts, etc., can solve the problems of affecting the judgment of the detector, performance degradation, insufficient detection performance, etc., and achieve the effect of improving detection performance and reducing noise.
- Summary
- Abstract
- Description
- Claims
- Application Information
AI Technical Summary
Problems solved by technology
Method used
Image
Examples
Embodiment
[0042] A dense pedestrian detection method based on dense frame generation network, including two parts: dense pedestrian detection model training and dense pedestrian detection model testing.
[0043] figure 1 Shown is a block diagram of the training process of the dense pedestrian detection model of the present invention. The specific implementation mainly includes training network construction, lmdb training data generation, pedestrian frame aspect ratio statistics and calculation of expected values, formulation of learning strategies, backpropagation update weights, and model preservation and other steps. figure 2 Shown is a test flow diagram of the complex background pedestrian detection model of the present invention, which mainly includes the steps of test network construction and parameter setting, test model initialization, test image reading, test network forward calculation, test result output and storage, etc.
[0044] image 3 It is the network structure diagra...
PUM
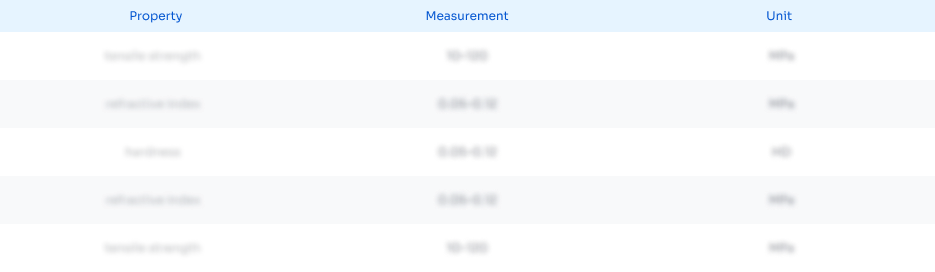
Abstract
Description
Claims
Application Information
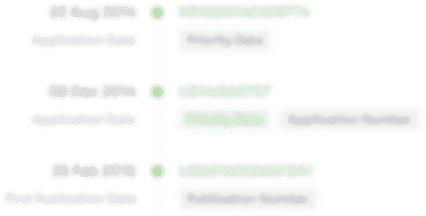
- Generate Ideas
- Intellectual Property
- Life Sciences
- Materials
- Tech Scout
- Unparalleled Data Quality
- Higher Quality Content
- 60% Fewer Hallucinations
Browse by: Latest US Patents, China's latest patents, Technical Efficacy Thesaurus, Application Domain, Technology Topic, Popular Technical Reports.
© 2025 PatSnap. All rights reserved.Legal|Privacy policy|Modern Slavery Act Transparency Statement|Sitemap|About US| Contact US: help@patsnap.com