Epileptic focus three-dimensional automatic positioning system based on deep learning
A deep learning and automatic positioning technology, applied in the field of medical imaging engineering, can solve problems such as false positives in dislocation areas, ignore subtle changes, and highly sensitive registration errors, achieving high accuracy and efficiency, consistent visual evaluation, and improved robustness sexual effect
- Summary
- Abstract
- Description
- Claims
- Application Information
AI Technical Summary
Problems solved by technology
Method used
Image
Examples
Embodiment Construction
[0040] The present invention will be described in further detail below in conjunction with the accompanying drawings and specific embodiments.
[0041] Such as figure 1 As shown, the three-dimensional automatic positioning system for epileptogenic focus in one embodiment of the present invention includes the following modules:
[0042] (1) PET image acquisition and labeling module, including image acquisition and epileptogenic focus area labeling:
[0043] 1.1) Acquisition of images: A 3D PET / CT scanner was used to acquire PET images of the brain. The subjects kept the same body position during the acquisition process to acquire PET images. Image format conversion is performed after image acquisition, that is, the original acquisition image sequence in DICOM format is converted into an image in NIFTI format that is easy to process.
[0044] 1.2) Mark samples: Divide the PET images into normal sample sets and sample sets with epileptogenic focus, and manually mark the epilept...
PUM
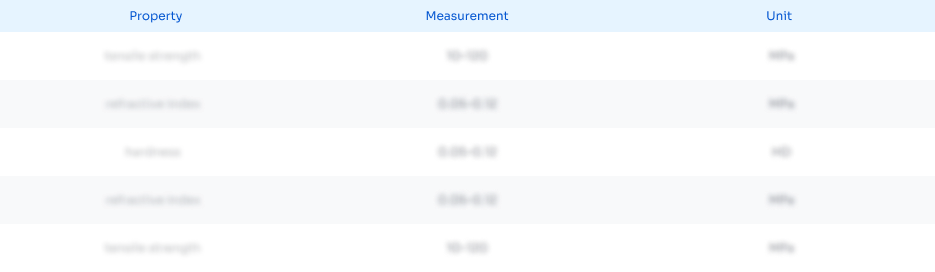
Abstract
Description
Claims
Application Information
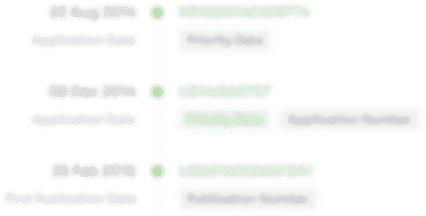
- R&D Engineer
- R&D Manager
- IP Professional
- Industry Leading Data Capabilities
- Powerful AI technology
- Patent DNA Extraction
Browse by: Latest US Patents, China's latest patents, Technical Efficacy Thesaurus, Application Domain, Technology Topic, Popular Technical Reports.
© 2024 PatSnap. All rights reserved.Legal|Privacy policy|Modern Slavery Act Transparency Statement|Sitemap|About US| Contact US: help@patsnap.com