Prognosis prediction system after liver transplantation
A prediction system and technology for liver transplantation, applied in diagnostic recording/measurement, biological neural network model, health index calculation, etc., can solve problems such as inaccurate research results, and achieve the goals of saving research costs, ensuring comprehensiveness, and improving accuracy Effect
- Summary
- Abstract
- Description
- Claims
- Application Information
AI Technical Summary
Problems solved by technology
Method used
Image
Examples
Embodiment 1
[0024] Basic as attached figure 1 Shown: the prognosis prediction system after liver transplantation, including the data acquisition module, used to obtain clinical data;
[0025] The database is used to store clinical data and establish data sets. Clinical data includes basic information of patients, clinical indicators, intraoperative treatment measures and prognosis after liver transplantation; during the process of collecting clinical data, in order to ensure the collected clinical Data validity, data screening criteria are stored in the database, and the data collection module collects clinical data according to the set data screening criteria;
[0026] The data correction module is used to organize and correct the collected clinical data; the data correction module is also used to fill in the missing values in the collected clinical data. Specifically, in this plan, the data correction module uses LOWESS local fitting interpolation The algorithm fills in the rows or c...
Embodiment 2
[0063] The difference from Embodiment 1 is that in this embodiment, the model construction module constructs a risk prediction model by combining a deep neural network and a random forest algorithm.
[0064]According to the characteristics of the data itself, considering the three stages of liver transplantation, namely, the diseased liver stage, the anhepatic stage, and the neoliver stage, due to the uneven frequency of various operations in the operation, the lack of many parameters, and the large number of Sparse indication parameters will cause the multi-layer deep neural network model to be almost impossible to learn sometimes. Therefore, in this embodiment, a combination of multi-layer deep neural network model and random forest is used to establish a risk prediction model for poor prognosis after liver transplantation. Carry out machine learning training; specifically, the above process is implemented in Python, and SQL is used for database construction. The computing s...
PUM
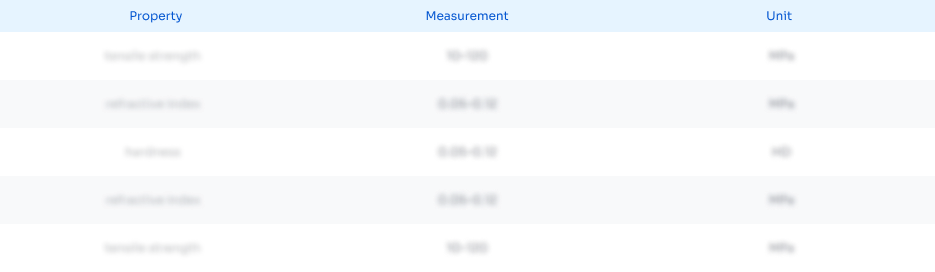
Abstract
Description
Claims
Application Information
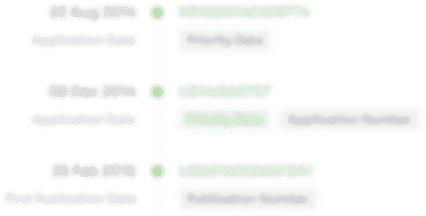
- R&D Engineer
- R&D Manager
- IP Professional
- Industry Leading Data Capabilities
- Powerful AI technology
- Patent DNA Extraction
Browse by: Latest US Patents, China's latest patents, Technical Efficacy Thesaurus, Application Domain, Technology Topic, Popular Technical Reports.
© 2024 PatSnap. All rights reserved.Legal|Privacy policy|Modern Slavery Act Transparency Statement|Sitemap|About US| Contact US: help@patsnap.com