Electric vehicle lithium ion battery health state estimation method based on AdaBoost-CBP neural network
A BP neural network, lithium-ion battery technology, applied in biological neural network models, neural architecture, computing, etc.
- Summary
- Abstract
- Description
- Claims
- Application Information
AI Technical Summary
Problems solved by technology
Method used
Image
Examples
Embodiment Construction
[0068] Taking the data subset of the No. 5 battery in the NASA public data set shown in Table 1 as an example, the technical solutions in the embodiments of the present invention are clearly and completely described in combination with the drawings in the embodiments of the present invention.
[0069] Embodiments of the present invention are described in detail below, examples of which are shown in the drawings, wherein the same or similar reference numerals designate the same or similar elements or elements having the same or similar functions throughout. The embodiments described below by referring to the figures are exemplary only for explaining the present invention and should not be construed as limiting the present invention.
[0070] Such as figure 1 As shown, according to an embodiment of the present invention, the method for estimating the state of health of an electric vehicle lithium-ion battery based on the AdaBoost-CBP neural network includes three basic steps: da...
PUM
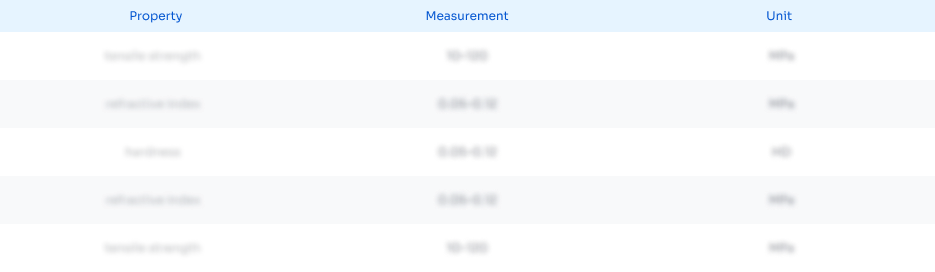
Abstract
Description
Claims
Application Information
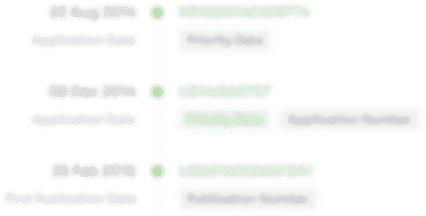
- R&D
- Intellectual Property
- Life Sciences
- Materials
- Tech Scout
- Unparalleled Data Quality
- Higher Quality Content
- 60% Fewer Hallucinations
Browse by: Latest US Patents, China's latest patents, Technical Efficacy Thesaurus, Application Domain, Technology Topic, Popular Technical Reports.
© 2025 PatSnap. All rights reserved.Legal|Privacy policy|Modern Slavery Act Transparency Statement|Sitemap|About US| Contact US: help@patsnap.com