Ubiquitous power Internet of Things perception data missing restoration method based on matrix filling
A power Internet of Things and sensing data technology, which is applied in the field of ubiquitous power Internet of Things sensing data missing repair based on matrix filling, and can solve problems such as consuming a lot of computing resources.
- Summary
- Abstract
- Description
- Claims
- Application Information
AI Technical Summary
Problems solved by technology
Method used
Image
Examples
Embodiment 1
[0034] In order to overcome the above problems, an embodiment of the present invention proposes a method for complementing missing power quality data based on low-rank matrix theory. The low-rank matrix theory restores missing data based on the low-rank nature of the data itself, thus avoiding the data pre-training process.
[0035] By analyzing the inherent characteristics of typical power quality data, it is proved that it has data low-rank recoverability; on this basis, considering the mixed noise and peak abnormal values of actual measurement data, a multi-norm joint low-rank optimization model is designed, and based on alternating The direction multiplier method is used to solve the model; at the same time, to solve the problem of slow model iteration, an adaptive iterative step selection method is proposed; finally, the effect of the algorithm is verified by analyzing the data recovery effect in high-frequency fault scenarios.
[0036] First, the measured one-dimension...
Embodiment 2
[0041] The scheme in embodiment 1 is further introduced below in conjunction with specific calculation formulas and examples, see the following description for details:
[0042] 1) Original sampling data reconstruction
[0043] The original sampling data are all one-dimensional time-domain sequences, which cannot be directly recovered, so they need to be organized into a matrix form.
[0044] Without loss of generality, it is assumed that a measuring point in the power grid collects data of n consecutive cycles, and each cycle f c subsampling. For the original data l, the slice transformation method is used to reconstruct it from a one-dimensional sequence in the time domain into a matrix form. The specific way is to follow the quarter cycle as the unit figure 1 The method organizes the data into a measurement data matrix L. Obviously, the size of the matrix L is related to the sampling time and sampling rate, which is 0.25f c ×4n order; at the same time, the transformati...
specific example
[0153] This section tests the effect of the recovery algorithm through the Real-life Power Quality Transients public data set. Select high-frequency fault scenarios: data under voltage swell, voltage sag, voltage interruption, pulse oscillation, and harmonic pollution. 50 cycles of data are extracted for each type of fault, and the data sampling rate is 20kHz, so there are 20,000 data points in total. At the same time, considering that random missing data will lead to fluctuations in the recovery effect, all results are obtained by taking the average value after repeating the experiment 30 times. Respectively verify the restoration effect of the singular value threshold algorithm SVT, the alternating direction multiplier method ADMM and the improved alternating direction multiplier method IADMM algorithm proposed by the present invention; on this basis, intercept a section with a 50% data missing rate, and observe the missing data recovery accuracy statistical distribution. ...
PUM
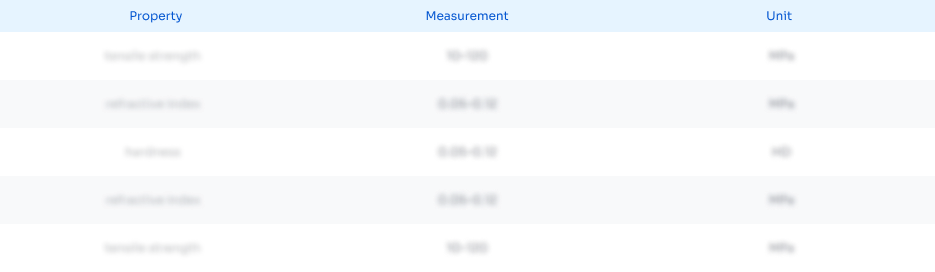
Abstract
Description
Claims
Application Information
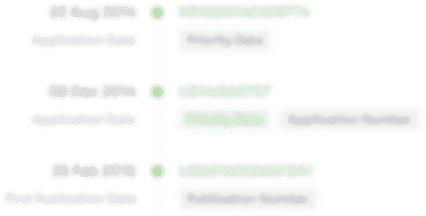
- R&D
- Intellectual Property
- Life Sciences
- Materials
- Tech Scout
- Unparalleled Data Quality
- Higher Quality Content
- 60% Fewer Hallucinations
Browse by: Latest US Patents, China's latest patents, Technical Efficacy Thesaurus, Application Domain, Technology Topic, Popular Technical Reports.
© 2025 PatSnap. All rights reserved.Legal|Privacy policy|Modern Slavery Act Transparency Statement|Sitemap|About US| Contact US: help@patsnap.com