Sample clustering and feature recognition method based on integrated non-negative matrix factorization
A non-negative matrix factorization and feature recognition technology, applied in the field of pattern recognition
- Summary
- Abstract
- Description
- Claims
- Application Information
AI Technical Summary
Problems solved by technology
Method used
Image
Examples
Embodiment Construction
[0033] With the implementation and completion of large-scale sequencing projects, massive omics data have been generated, which has brought great challenges to researchers' analysis and calculation. Therefore, the development of efficient multi-omics data processing methods has important theoretical significance and application value.
[0034] Due to the limitation of experimental conditions, experimental samples are usually only dozens to hundreds, and sequencing technology can monitor tens of thousands of genes at the same time. Therefore, the primary challenge in analyzing multi-omics data is that the dimensionality of data features is much higher than the number of samples. In addition, realistic multi-omics data contains a lot of noise and redundant information; different types of data from different platforms need to be processed simultaneously, such as count data from sequencing, continuous data from microarrays, binary data from genetic variation These are all issues t...
PUM
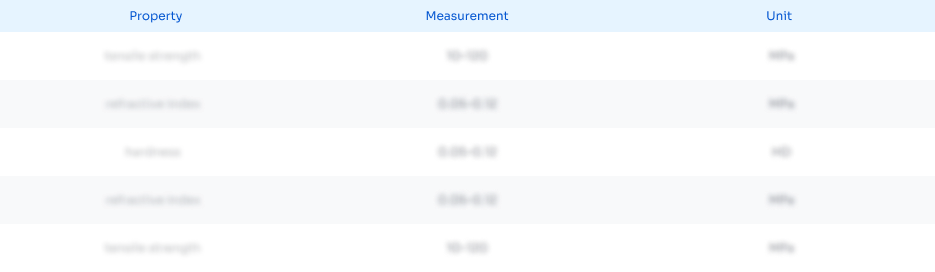
Abstract
Description
Claims
Application Information
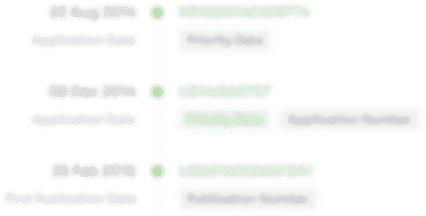
- R&D Engineer
- R&D Manager
- IP Professional
- Industry Leading Data Capabilities
- Powerful AI technology
- Patent DNA Extraction
Browse by: Latest US Patents, China's latest patents, Technical Efficacy Thesaurus, Application Domain, Technology Topic, Popular Technical Reports.
© 2024 PatSnap. All rights reserved.Legal|Privacy policy|Modern Slavery Act Transparency Statement|Sitemap|About US| Contact US: help@patsnap.com