Electrocardiosignal classification method and device, electronic equipment and storage medium
A technology of electrocardiographic signal and classification method, applied in character and pattern recognition, instrument, biological neural network model, etc., can solve problems such as huge labeling cost and huge size
- Summary
- Abstract
- Description
- Claims
- Application Information
AI Technical Summary
Problems solved by technology
Method used
Image
Examples
Embodiment 1
[0092] figure 1 A flowchart of a method for classifying ECG signals provided in Embodiment 1, according to figure 1 As shown, the ECG signal classification method in Embodiment 1 includes the following steps:
[0093] Step S101: Acquire ECG signals.
[0094] The electrocardiographic signal is the signal of the electrical activity change generated by the heart during each cardiac cycle recorded from the body surface, and is usually recorded graphically through an electrocardiogram (ECG). In the embodiment of the present application, multi-channel synchronous data may be used to collect and store human heart signals, background noise, and electrocardiographic signals. For example, electrocardiographic signals can be collected through electrocardiographic leads and sensors, and analog signals of human physiological parameters can be converted into digital signals by analog-to-digital converters, which are stored in memory.
[0095] like figure 2 As shown, in an example, afte...
Embodiment 2
[0123] Figure 5 A flowchart of a method for classifying electrocardiographic signals provided in Embodiment 2, according to Figure 5 As shown, the electrocardiographic signal classification method in the second embodiment includes the following steps:
[0124] Step S501: Obtain ECG signals.
[0125] Step S502: Detecting the QRS complex wave from the electrocardiographic signal.
[0126] Specifically, such as Image 6 As shown, the described detection of the QRS complex from the electrocardiographic signal may include the following sub-steps:
[0127] Step S5021: Perform discrete wavelet decomposition on the ECG signal to obtain a preset number of wavelet decomposition coefficients.
[0128] In an example, Daubechies4 (Db4) wavelet may be used to perform discrete wavelet decomposition on the preprocessed ECG signal. The wavelet decomposition coefficients are determined by the number of layers of wavelet decomposition. In a preferred example, the number of wavelet decompo...
Embodiment 3
[0172] Figure 9 A flow chart of a method for classifying electrocardiographic signals provided in Embodiment 3, according to Figure 9 As shown, the electrocardiographic signal classification method in the third embodiment includes the following steps:
[0173] Step S901: Acquiring ECG signals.
[0174] Step S902: Detecting the QRS complex wave from the electrocardiographic signal.
[0175] Step S903: Cutting the electrocardiographic signal from which the QRS complex wave is detected into a single heart beat, and packaging the cut heart beat signal.
[0176] Step S904: Input the plurality of cardiac beat packets into the second deep network model;
[0177] Step S905: Using the second deep network model to extract features of each heartbeat in the plurality of heartbeat packets, and input the extracted features to the support vector machine.
[0178] Step S906: Using the support vector machine to classify the features of each heartbeat, and obtain the type identification r...
PUM
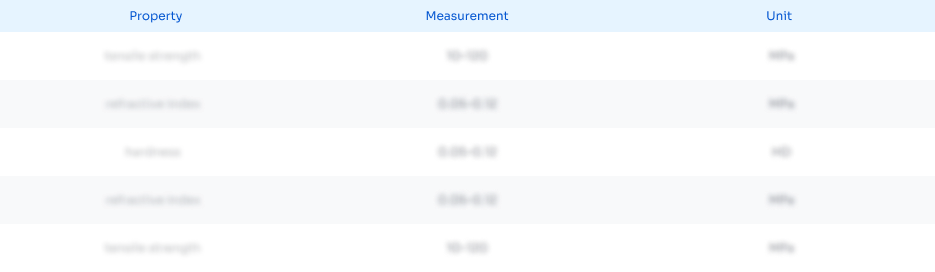
Abstract
Description
Claims
Application Information
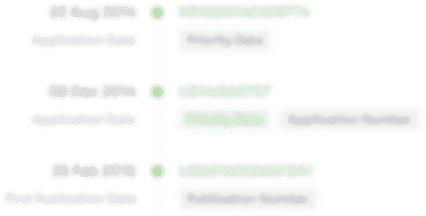
- R&D Engineer
- R&D Manager
- IP Professional
- Industry Leading Data Capabilities
- Powerful AI technology
- Patent DNA Extraction
Browse by: Latest US Patents, China's latest patents, Technical Efficacy Thesaurus, Application Domain, Technology Topic, Popular Technical Reports.
© 2024 PatSnap. All rights reserved.Legal|Privacy policy|Modern Slavery Act Transparency Statement|Sitemap|About US| Contact US: help@patsnap.com