Textile crease detection method based on deep learning
A technology of deep learning and textiles, applied in neural learning methods, image data processing, instruments, etc., can solve the problems of manual design parameters such as heavy workload, easy to be affected by uneven illumination, and low system security, so as to reduce manual adjustment. parameters, improve convenience, and improve detection efficiency
- Summary
- Abstract
- Description
- Claims
- Application Information
AI Technical Summary
Problems solved by technology
Method used
Image
Examples
Embodiment 1
[0051] A method for detecting creases in textiles based on deep learning, the method comprising:
[0052] (1) Extract the textile creases from the original image of the textile.
[0053] When the camera used is an RGB camera, the image needs to be grayscaled. This can be undone if using a grayscale camera. Assuming that the image S is a grayscale image, the corresponding pixel should have S(i,j)=max(R(i,j),G(i,j),B(i,j)), where R(i ,j), G(i,j), and B(i,j) are the data of the R, G, and B channels of the original textile image respectively, and the original grayscale image S(i,j) of the textile is obtained.
[0054] (1a) Perform histogram equalization processing on the original grayscale image of the textile.
[0055] In order to perform self-quotient processing on the image, it is necessary to filter the spatial domain of the grayscale image to enhance the contrast with the original image, so that the self-quotient effect is better. First, histogram equalization is performed ...
PUM
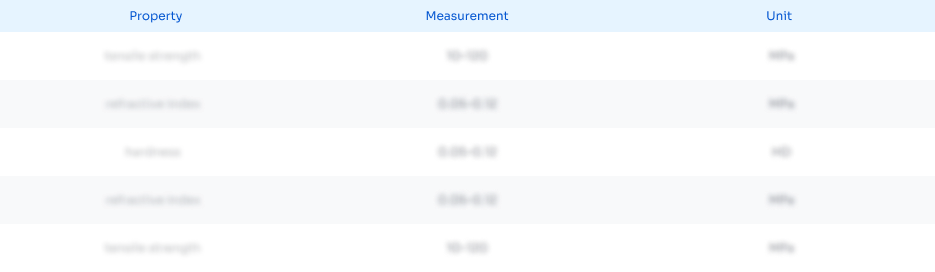
Abstract
Description
Claims
Application Information
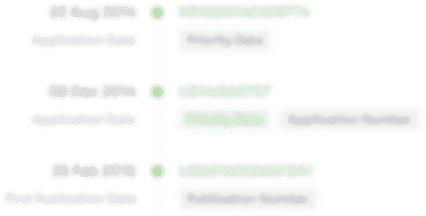
- R&D
- Intellectual Property
- Life Sciences
- Materials
- Tech Scout
- Unparalleled Data Quality
- Higher Quality Content
- 60% Fewer Hallucinations
Browse by: Latest US Patents, China's latest patents, Technical Efficacy Thesaurus, Application Domain, Technology Topic, Popular Technical Reports.
© 2025 PatSnap. All rights reserved.Legal|Privacy policy|Modern Slavery Act Transparency Statement|Sitemap|About US| Contact US: help@patsnap.com