Fault diagnosis method for rolling bearing under variable working conditions
A technology for fault diagnosis and variable working conditions, which is applied in neural learning methods, detecting faulty computer hardware, and using neural networks to detect faulty hardware. It can solve problems such as model generality and generalization deterioration.
- Summary
- Abstract
- Description
- Claims
- Application Information
AI Technical Summary
Problems solved by technology
Method used
Image
Examples
Embodiment Construction
[0064] The specific implementation manners of the present invention will be further described in detail below in conjunction with the accompanying drawings and embodiments. The following examples are used to illustrate the present invention, but are not intended to limit the scope of the present invention.
[0065] Below in conjunction with actual experimental data the present invention is described in detail:
[0066] The experimental data adopts the bearing data set of Case Western Reserve University. The data acquisition system consists of three main parts: motor, torque sensor and dynamometer. Accelerometers are used to collect vibration data, and the sampling frequency is 12KHz. Faults are introduced in rollers, inner and outer rings by electric discharge machining (EDM), and different fault sizes are set.
[0067] Such as figure 1 Shown, the present invention comprises the following steps:
[0068] Step 1: Collect vibration data of bearings in different health states ...
PUM
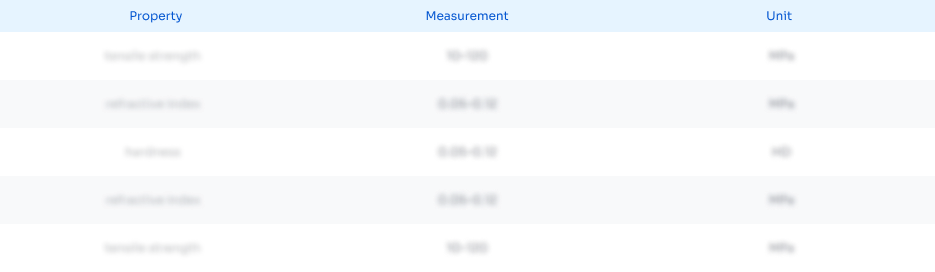
Abstract
Description
Claims
Application Information
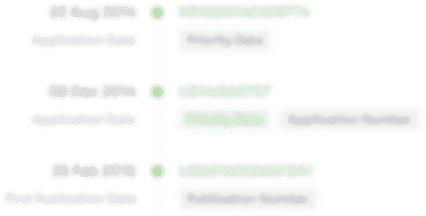
- R&D Engineer
- R&D Manager
- IP Professional
- Industry Leading Data Capabilities
- Powerful AI technology
- Patent DNA Extraction
Browse by: Latest US Patents, China's latest patents, Technical Efficacy Thesaurus, Application Domain, Technology Topic, Popular Technical Reports.
© 2024 PatSnap. All rights reserved.Legal|Privacy policy|Modern Slavery Act Transparency Statement|Sitemap|About US| Contact US: help@patsnap.com