MRI brain tumor automatic segmentation method of double-flow decoding convolutional neural network based on edge feature optimization
A convolutional neural network and edge feature technology, applied in the field of MRI brain tumor automatic segmentation, can solve the problems of not paying attention to the edge part features and segmentation effect, affecting the accuracy of tumor segmentation, and not fully utilizing the deep learning of the network, reaching the edge The effect of feature enhancement
- Summary
- Abstract
- Description
- Claims
- Application Information
AI Technical Summary
Problems solved by technology
Method used
Image
Examples
Embodiment Construction
[0027] figure 2 It is represented as an algorithm model diagram of the deep convolutional neural network used in the present invention. The input of the algorithm is MR images containing four modalities, including four weighted images of T1, T1c, T2, and Flair. The model includes encoding network for feature extraction, edge stream decoding network, semantic stream decoding network, edge feature processing module and feature fusion layer. Among them, the edge feature processing module and the feature fusion layer form the edge feature fusion module, and the edge stream decoding network, semantic stream decoding network and edge feature fusion module form the dual-stream decoding network. Specifically, the encoding network behaves as figure 2 The continuous downsampling network part on the left side of the middle, the edge stream decoding network is shown as figure 2 The continuous upsampling network in the middle part, the semantic flow decoding network is shown as fig...
PUM
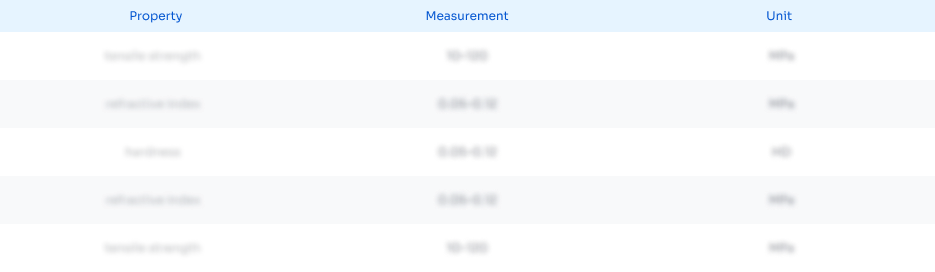
Abstract
Description
Claims
Application Information
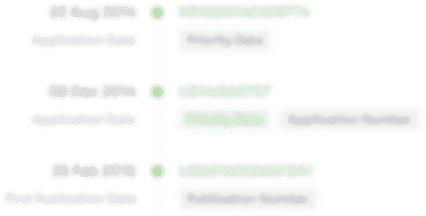
- R&D Engineer
- R&D Manager
- IP Professional
- Industry Leading Data Capabilities
- Powerful AI technology
- Patent DNA Extraction
Browse by: Latest US Patents, China's latest patents, Technical Efficacy Thesaurus, Application Domain, Technology Topic, Popular Technical Reports.
© 2024 PatSnap. All rights reserved.Legal|Privacy policy|Modern Slavery Act Transparency Statement|Sitemap|About US| Contact US: help@patsnap.com