Method for inverting simulation parameters through machine learning neural network
A neural network and machine learning technology, applied in the field of inversion of simulation parameters through machine learning neural network, can solve problems such as simulation result deviation, error numerical model, error programming, etc., to reduce simulation errors and minimize differences.
- Summary
- Abstract
- Description
- Claims
- Application Information
AI Technical Summary
Problems solved by technology
Method used
Image
Examples
Embodiment 1
[0039] see Figure 1 to Figure 8 , a method for inverting simulation parameters through a machine learning neural network, comprising the following steps:
[0040] S1, establish a machine learning neural network, including an input layer and an output layer, the test conditions of the input layer and the output layer are the same as those of the physical model;
[0041] S2, use a simple physical model as the basis for simulation prediction, simulating multiple possible working conditions of the injection molding process of the designed plastic parts; this embodiment simulates 18 working conditions of its plastic parts, and the input data is as follows image 3 shown;
[0042] S3. In the machine learning neural network and physical model, input the same original parameters according to the injection molding process, and use the physical model as the learning object to invert and adjust the operation of the machine learning neural network to match the third weight set W3 , min...
Embodiment 2
[0054] This embodiment specifically describes the training of the machine learning neural network model. The difference from the above embodiment is that the rheological model parameters of the recycled polypropylene plastic corresponding to the original plastic in Example 1 are reversed. The rheological model parameters in this embodiment Specifically, model parameters include at least one set of shear viscosity model parameters and a set of additional model parameters for calculating pressure loss in case of constricted flow. This additional model requires that a pressure transducer be placed before and after the systolic flow to measure the pressure loss of the systolic flow. Additional model parameters can be better measured by designing and using at least two or more different constricted flows.
[0055] On measurement data, two forms are acceptable:
[0056] 1) A constant value at a certain moment, for example, the moment corresponding to the peak value measured by one ...
Embodiment 3
[0081] Propose a spiral mold in this embodiment, the physical model of this embodiment is injection mold flow analysis software, also can be the physical model of any injection mold flow analysis in other embodiments, use three melt temperature conditions equally, be respectively 220 °C, 240 °C, and 260 °C have corresponding speed and pressure values at each melt temperature, and the specific values are in Figure 10 listed in . The material used for the experiments was polypropylene Stamylan PHC 31, as Figure 11 Shown is a mold flow analysis of the spiral plastic part used, a simulation of the spiral mold was performed to provide a predictive equation based on a second order viscosity model as follows:
[0082]
[0083] Wherein η is viscosity (Pa.s); A i model coefficients; Shear rate (1 / s); T temperature (°C).
[0084] Similarly, in this embodiment, the second-order viscosity model is used as the training model of the neural network model of the present inventio...
PUM
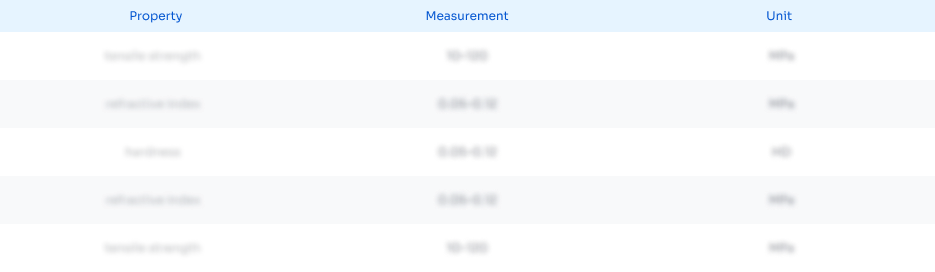
Abstract
Description
Claims
Application Information
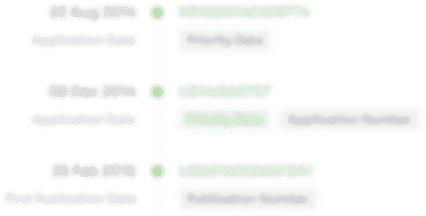
- R&D Engineer
- R&D Manager
- IP Professional
- Industry Leading Data Capabilities
- Powerful AI technology
- Patent DNA Extraction
Browse by: Latest US Patents, China's latest patents, Technical Efficacy Thesaurus, Application Domain, Technology Topic, Popular Technical Reports.
© 2024 PatSnap. All rights reserved.Legal|Privacy policy|Modern Slavery Act Transparency Statement|Sitemap|About US| Contact US: help@patsnap.com