Alzheimer's disease classification method based on improved 3D CNN network
A technology of Alzheimer's disease and classification method, which is applied in the field of Alzheimer's disease classification, can solve the problems affecting model accuracy and difficulty in model training, and achieve the effects of improving accuracy, easy training, and solving model degradation problems
- Summary
- Abstract
- Description
- Claims
- Application Information
AI Technical Summary
Problems solved by technology
Method used
Image
Examples
Embodiment
[0071] The constructed model structure and parameters are shown in Table 1. Except for the first module, other backbone structures maintain the original structure of VGG, that is, the convolution kernel size is 3×3×3, the step size is 1, and the edge is zero-filled to Leave the output size unchanged. Divide VGG into a MaxPooling layer of 6 modules (Block), whose kernel size is set to 2×2×2, and the step size is 2 to realize the downsampling operation. When the value is too large or too small, the derivatives of activation functions such as Sigmoid and Tanh are close to 0, while ReLU is an unsaturated activation function, which does not exist, and its derivation is easier to calculate, making network training faster, so it is very The linear activation layer uses the ReLU activation function. The detailed parameters of the six VGG modules divided by Max-Pooling are shown in Table 1, where 3DConvolution includes Conv3d layer, BatchNorm3d layer, ReLU layer, Skip Connection inclu...
PUM
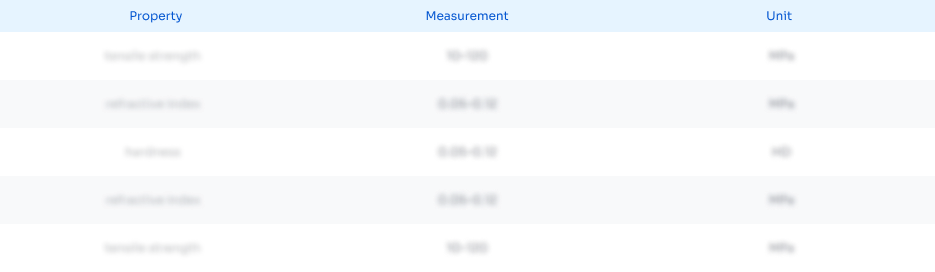
Abstract
Description
Claims
Application Information
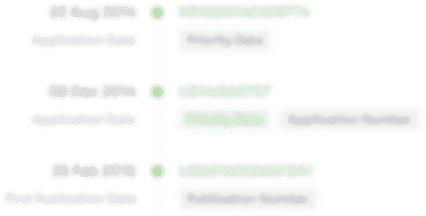
- R&D
- Intellectual Property
- Life Sciences
- Materials
- Tech Scout
- Unparalleled Data Quality
- Higher Quality Content
- 60% Fewer Hallucinations
Browse by: Latest US Patents, China's latest patents, Technical Efficacy Thesaurus, Application Domain, Technology Topic, Popular Technical Reports.
© 2025 PatSnap. All rights reserved.Legal|Privacy policy|Modern Slavery Act Transparency Statement|Sitemap|About US| Contact US: help@patsnap.com