Active learning method based on covariance matrix
A covariance matrix and active learning technology, applied in the field of machine learning, can solve problems such as difficulty in obtaining sample information, waste of data resources, difficulty in achieving ideal results in model prediction accuracy and generalization ability, and achieve high-quality results
- Summary
- Abstract
- Description
- Claims
- Application Information
AI Technical Summary
Problems solved by technology
Method used
Image
Examples
Embodiment 1
[0046] refer to figure 1 , provides a schematic diagram of the overall structure of the active learning method based on the covariance matrix, such as figure 1, an active learning method based on the covariance matrix includes collecting the butane concentration value in the debutanizer production process, and using the butane concentration value as a soft sensor modeling sample; dividing the soft sensor modeling sample into a training set and a test set , the training set is divided into labeled sample set and unlabeled sample set; the Gaussian process regression model is established by using the labeled sample set, and the initial parameters of the model are determined; according to the unlabeled sample set, the largest determinant of the covariance matrix is selected. samples; re-establish the Gaussian process regression model, determine the model parameters; use the test set to predict the concentration of butane.
[0047] The parameters of the Gaussian process regressi...
Embodiment 2
[0085] In this embodiment, the active learning modeling method based on the covariance matrix proposed by the present invention is compared with the traditional Gaussian process regression active learning algorithm based on the prediction variance selection.
[0086] In order to test the difference between the two selection strategies for the selection of unlabeled samples, a regression analysis is performed on the function z=sin(2x)+cos(4y), where x and y are all subject to normal distribution; the data set is divided into 20 groups. Labeled sample sets, 400 sets of unlabeled sample sets, and 400 sets of test sample sets;
[0087] Specific process: select 5 and 10 unlabeled samples respectively for labeling; in the third iteration, the selection results of unlabeled samples are shown in Figure 4.
[0088] In Figure 4, the yellow points are the distribution points of the unlabeled sample set, the green points are the unlabeled samples selected based on the variance selection s...
Embodiment 3
[0091] In order to monitor the refining quality, it is necessary to monitor the butane concentration at the bottom of the column in real time; however, it is difficult to directly detect the outflow of the bottom material of the debutanizer, and a soft sensor model needs to be established.
[0092] During the real-time sampling process of the butane concentration in the debutanizer at the No. 81 natural gas processing station of the No. Contains only 7 process variables; 20 unlabeled samples are selected each time and added to the labeled sample set, and another 500 groups of samples are selected as test samples.
[0093] In order to verify the effectiveness of the unlabeled sample selection strategy proposed by the present invention, it is compared with the variance-based selection and random sample selection strategies.
[0094]The random sample selection is to randomly select 20 samples from the unlabeled sample set each time, and add the labeled sample set after marking to...
PUM
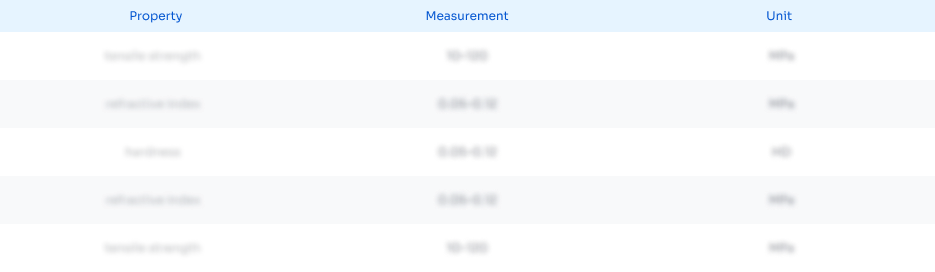
Abstract
Description
Claims
Application Information
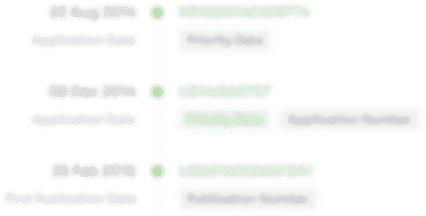
- R&D
- Intellectual Property
- Life Sciences
- Materials
- Tech Scout
- Unparalleled Data Quality
- Higher Quality Content
- 60% Fewer Hallucinations
Browse by: Latest US Patents, China's latest patents, Technical Efficacy Thesaurus, Application Domain, Technology Topic, Popular Technical Reports.
© 2025 PatSnap. All rights reserved.Legal|Privacy policy|Modern Slavery Act Transparency Statement|Sitemap|About US| Contact US: help@patsnap.com