Service load fine-grained prediction method based on AKX hybrid model
A hybrid model and prediction method technology, applied in the field of service computing, can solve problems such as single model, ignoring a large amount of historical data for effective analysis and utilization, lack of effective real-time correction of prediction results, etc., to achieve the effect of ensuring availability
- Summary
- Abstract
- Description
- Claims
- Application Information
AI Technical Summary
Problems solved by technology
Method used
Image
Examples
specific Embodiment 1
[0070] The present invention provides a service load fine-grained prediction method based on the AKX hybrid model, specifically:
[0071] A method for fine-grained forecasting of service load based on the AKX hybrid model, comprising the following steps:
[0072] step 1:
[0073] Collect online real-time data generated during service operation monitoring, and perform data preprocessing on the collected service load data to obtain online real-time service load data sets;
[0074] The step 1 is specifically:
[0075] Collect service load data during the service operation monitoring process, including CPU utilization and memory utilization, and perform data preprocessing on the collected CPU utilization and memory utilization, remove the noise in the data, and finally obtain the service load data set.
[0076] Step 2: Perform white noise detection on the collected service load data set to ensure the availability of the service load data set;
[0077] The step 2 is specificall...
specific Embodiment 2
[0126] From figure 1 It can be seen that with the method provided by the present invention, online real-time service load prediction and correction based on Kalman will be performed from multiple perspectives, as well as XGBoost offline prediction correction using historical service load data. First, the service load will be online through the time series ARIMA model. Real-time service load prediction, and then input the service load prediction value based on the ARIMA model into Kalman for forecast correction, considering the value of historical service load data and the data accuracy problem caused by the difference calculation of the unstationary sequence in the data preprocessing process, and ARIMA For the residual problem caused by the nonlinear part in the model, XGBoost autoregressive machine learning will be performed on the service load historical data offline to more comprehensively correct the online real-time service load prediction based on the ARIMA-Kalman model, ...
PUM
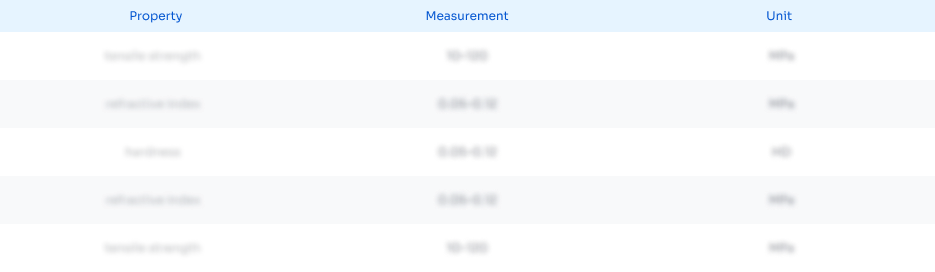
Abstract
Description
Claims
Application Information
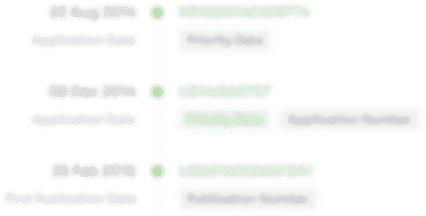
- R&D
- Intellectual Property
- Life Sciences
- Materials
- Tech Scout
- Unparalleled Data Quality
- Higher Quality Content
- 60% Fewer Hallucinations
Browse by: Latest US Patents, China's latest patents, Technical Efficacy Thesaurus, Application Domain, Technology Topic, Popular Technical Reports.
© 2025 PatSnap. All rights reserved.Legal|Privacy policy|Modern Slavery Act Transparency Statement|Sitemap|About US| Contact US: help@patsnap.com