Three-dimensional particle category detection method and system based on convolutional neural network
A convolutional neural network and detection method technology, applied in the field of structural biology cryo-electron tomography, can solve the problems of lack of automation of three-dimensional particles and excessive model parameters
- Summary
- Abstract
- Description
- Claims
- Application Information
AI Technical Summary
Problems solved by technology
Method used
Image
Examples
Embodiment Construction
[0070] When the inventors researched the selection and classification methods of three-dimensional particles in cryo-electron tomography reconstruction, they found that there are three problems in the existing methods. First, existing work generally lacks automated processing procedures. When the input electronic tomographic reconstruction is collected by different imaging parameters and contains different types of particles, the existing methods need to adjust all interaction parameters according to the properties of the data to achieve the best effect by manual intervention. Second, existing work has not yet addressed the high-noise situation of frozen electronic data. When the signal-to-noise ratio of the input electron tomographic reconstruction image is very low, the performance of the existing methods may be affected. Third, methods based on deep learning have the disadvantage of large model parameters. This kind of network training is difficult, takes a long time, req...
PUM
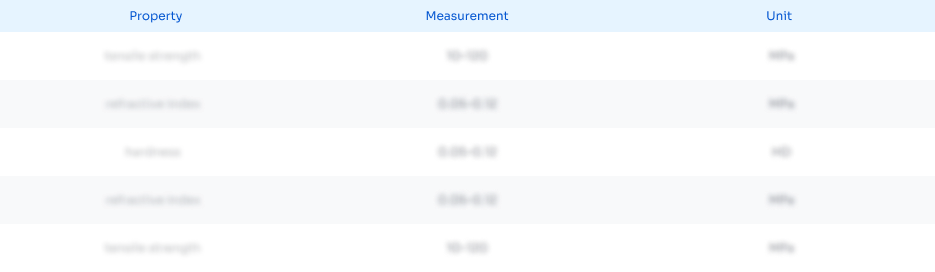
Abstract
Description
Claims
Application Information
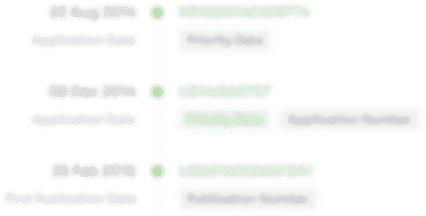
- R&D Engineer
- R&D Manager
- IP Professional
- Industry Leading Data Capabilities
- Powerful AI technology
- Patent DNA Extraction
Browse by: Latest US Patents, China's latest patents, Technical Efficacy Thesaurus, Application Domain, Technology Topic, Popular Technical Reports.
© 2024 PatSnap. All rights reserved.Legal|Privacy policy|Modern Slavery Act Transparency Statement|Sitemap|About US| Contact US: help@patsnap.com