cnn accelerator and electronics
An accelerator and engine technology, applied in the field of convolutional neural network, can solve problems such as time cost, waste of memory resources, inflexibility, etc., and achieve the effects of reducing inference error rate, good scalability, and improving performance
- Summary
- Abstract
- Description
- Claims
- Application Information
AI Technical Summary
Problems solved by technology
Method used
Image
Examples
Embodiment Construction
[0031] The technical solutions proposed by the present invention will be described in further detail below in conjunction with the accompanying drawings and specific embodiments. The advantages and features of the present invention will become clearer from the following description. It should be noted that all the drawings are in a very simplified form and use imprecise scales, and are only used to facilitate and clearly assist the purpose of illustrating the embodiments of the present invention. In this article, "and / or" means to choose one or both.
[0032] Please refer to figure 1 and figure 2 , an embodiment of the present invention proposes a CNN accelerator based on MCU core 11 and FPGA core 12 System-on-Chip (SoC) 1, this SoC1 takes MCU core 11 as the core, based on FPGA core 12 programmable characteristics, realizes the CNN accelerator The training phase and the inference phase of a convolutional neural network. Specifically, the CNN accelerator includes a CNN tra...
PUM
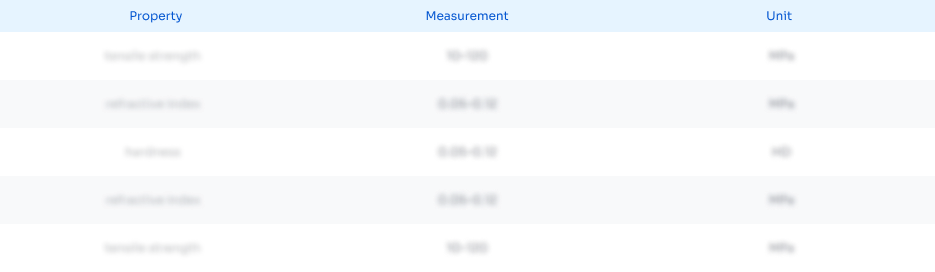
Abstract
Description
Claims
Application Information
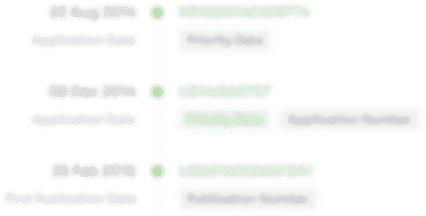
- Generate Ideas
- Intellectual Property
- Life Sciences
- Materials
- Tech Scout
- Unparalleled Data Quality
- Higher Quality Content
- 60% Fewer Hallucinations
Browse by: Latest US Patents, China's latest patents, Technical Efficacy Thesaurus, Application Domain, Technology Topic, Popular Technical Reports.
© 2025 PatSnap. All rights reserved.Legal|Privacy policy|Modern Slavery Act Transparency Statement|Sitemap|About US| Contact US: help@patsnap.com