Regional network flow prediction method based on deep learning
A technology for regional network and traffic prediction, which is applied in the field of regional network traffic prediction based on deep learning, and can solve the problems of not covering the periodic variability of traffic, low accuracy, and not considering the spatial correlation of regional traffic prediction.
- Summary
- Abstract
- Description
- Claims
- Application Information
AI Technical Summary
Problems solved by technology
Method used
Image
Examples
Embodiment Construction
[0075] In order to describe the technical solution disclosed in the present invention in detail, further elaboration will be made below in conjunction with the accompanying drawings and specific embodiments.
[0076] The invention provides a method for predicting regional traffic based on deep learning. Aiming at the temporal correlation and spatial correlation of regional traffic, the spatio-temporal features are simultaneously extracted through 3D convolutional neural network and ConvLSTM. The method of time series extraction enables the forecasting network to learn the periodic change characteristics of the traffic sequence under the limited input sequence length. Finally, the final regional traffic prediction result is obtained through the multi-layer perceptron.
[0077] Step 1: Obtain the regional network traffic sequence, and count the traffic value used by it at each moment.
[0078] (1) Divide the network coverage area into N×M grid areas of 1km×1km, record the coor...
PUM
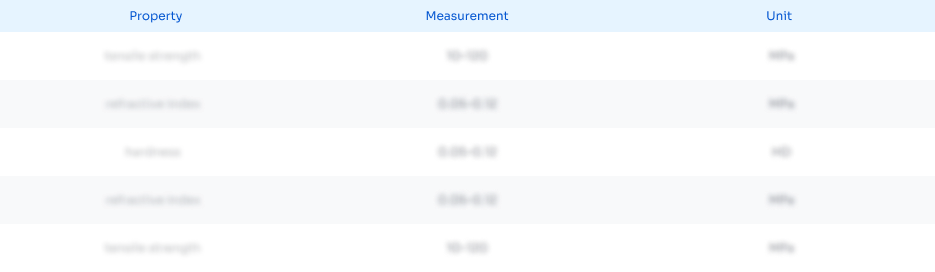
Abstract
Description
Claims
Application Information
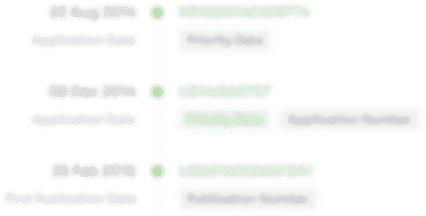
- R&D
- Intellectual Property
- Life Sciences
- Materials
- Tech Scout
- Unparalleled Data Quality
- Higher Quality Content
- 60% Fewer Hallucinations
Browse by: Latest US Patents, China's latest patents, Technical Efficacy Thesaurus, Application Domain, Technology Topic, Popular Technical Reports.
© 2025 PatSnap. All rights reserved.Legal|Privacy policy|Modern Slavery Act Transparency Statement|Sitemap|About US| Contact US: help@patsnap.com