Image detection method based on ultrasonic imaging and Bayesian optimization
A technology of ultrasonic imaging detection and ultrasonic imaging, which is applied in the directions of ultrasonic/sonic/infrasonic image/data processing, organ movement/change detection, ultrasonic/sonic/infrasonic diagnosis, etc. Infection and other issues
- Summary
- Abstract
- Description
- Claims
- Application Information
AI Technical Summary
Problems solved by technology
Method used
Image
Examples
Embodiment 1
[0077] The concrete steps of described step S6 are as follows:
[0078] S61: Initialization of model hyperparameters: Bayesian optimization KPCANet model hyperparameters initialization, initialization parameters mainly include the first layer filter size PatchSize1, the number of first layer filters NumFilters1, the second layer filter size PatchSize2, the first layer The number of filters NumFilters2, the local histogram block size HistBlockSize1, HistBlockSize2 and the overlapping block area ratio BlockOverLapRatio;
[0079] S62: Model performance calculation: According to the initialized model hyperparameters, determine them as the initialization parameters of the KPCANet model, and then input the training set samples into the model for training of the analysis model. After the model is trained, use the verification set samples for preliminary verification The performance of the model, and record the detection error rate E of the verification set;
[0080] S63: Bayesian al...
Embodiment 2
[0089] In described step S62, utilize KPCANet model to miner's lung ultrasonic imaging data analysis, its specific steps are as follows:
[0090] S621: Construct the first layer of KPCA;
[0091] S622: Using the output of the first layer KPCA to construct the second layer KPCA;
[0092] S623: Perform binary hashing and block histogram processing;
[0093] S624: SVM classification of lung ultrasound imaging characteristics: input the features of the lung ultrasound imaging information extracted by the two-layer KPCANet into the SVM classifier, wherein the Gaussian function is selected as the kernel function of the SVM.
Embodiment 3
[0095] The step of constructing the first layer of KPCA in step S621 is as follows:
[0096] S6211: The preprocessed ultrasound image I i The pixels are divided into k 1 *k 2 The size of the image block, after Patch vectorization, all the point information is arranged in an orderly manner, which is recorded as
[0097] S6212: Then convert each image block matrix into a column vector and perform mean removal operation to obtain:
[0098]
[0099] S6213: Perform the above steps S5211 and S5212 on all input images to obtain:
[0100]
[0101] S6214: Calculate the input kernel matrix K 1 :
[0102] K 1 =ConstructKernelMatrix(X) (3)
[0103] and K 1 Center to get K c1 , the number of filters in the first layer is NumFilters1, the purpose of the KPCA algorithm is to extract K c1 The eigenvectors corresponding to the largest eigenvalues of the first NumFilters1 are used as convolution filters:
[0104]
[0105] The main information of these zero-mean training sa...
PUM
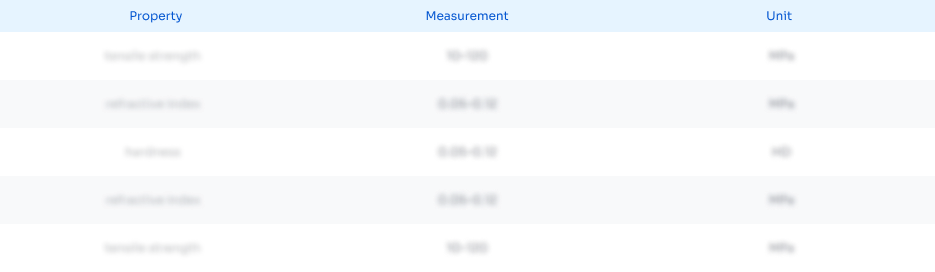
Abstract
Description
Claims
Application Information
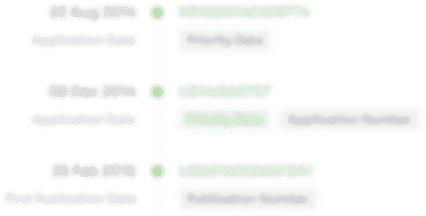
- R&D Engineer
- R&D Manager
- IP Professional
- Industry Leading Data Capabilities
- Powerful AI technology
- Patent DNA Extraction
Browse by: Latest US Patents, China's latest patents, Technical Efficacy Thesaurus, Application Domain, Technology Topic, Popular Technical Reports.
© 2024 PatSnap. All rights reserved.Legal|Privacy policy|Modern Slavery Act Transparency Statement|Sitemap|About US| Contact US: help@patsnap.com