Drug target interaction prediction method based on multi-channel graph convolutional network
A technology of convolution network and prediction method, which is applied in the field of prediction of the relationship between drugs and targets, and can solve problems such as poor accuracy of drug targets and inaccurate features
- Summary
- Abstract
- Description
- Claims
- Application Information
AI Technical Summary
Problems solved by technology
Method used
Image
Examples
specific Embodiment approach 1
[0029] Specific implementation mode 1. Combination figure 1 This embodiment will be described. A drug-target interaction prediction method based on a multi-channel graph convolutional network described in this embodiment, the method specifically includes the following steps:
[0030] Step 1. Extract drug information, protein information, disease information, and drug side effects information from the database, and construct a heterogeneous network based on the extracted information;
[0031] Then use the Jaccard similarity method and the random restart walk method to process the constructed heterogeneous network, and obtain the drug diffusion state matrix and protein diffusion state matrix;
[0032] Step 2, performing noise reduction and dimensionality reduction on the drug diffusion state matrix and the protein diffusion state matrix, respectively, to obtain the drug characteristic matrix and the protein characteristic matrix;
[0033] Step 3. Splice the drug feature matrix...
specific Embodiment approach 2
[0050] Specific embodiment 2: The difference between this embodiment and specific embodiment 1 is that in the first step, drug information, protein information, disease information, and drug side effects information are extracted from the database, and a heterogeneous network; its specific process is:
[0051] Extract drug information from the DrugBank database, the drug information includes drug-drug interaction information and known drug-target interaction information;
[0052] Extracting protein information from the HPRD database, the protein information is protein-protein interaction information;
[0053] Extracting disease information from the toxicogenomics database, the disease information including the relationship information between the disease and the drug and the relationship information between the disease and the protein;
[0054] Extract drug side effect information from the SIDER database, where the drug side effect information is the relationship information ...
specific Embodiment approach 3
[0058] Specific embodiment three: the difference between this embodiment and specific embodiment two is that in the first step, the Jaccard similarity method and the random restart walk method are used to process the constructed heterogeneous network to obtain the drug diffusion state matrix and protein Diffusion state matrix; the specific process is:
[0059] For the drug and drug side effect relationship network, the drug and drug side effect relationship network is expressed in the form of matrix C:
[0060]
[0061] Among them, c i′j′ = 0 or 1, c i′j′ = 1 means that the i′th drug is related to the side effect of the j′th drug, c i′j′ =0 means that the i'th drug has no relationship with the side effects of the j'th drug, i'=1,2,...,M, j'=1,2,...O;
[0062] Use the Jaccard similarity method to calculate the similarity between the i-th row and the j-th row of the matrix C, i=1, 2,..., M, j=1, 2,..., M, the calculated i-th row and j-th row The similarity of the row is u...
PUM
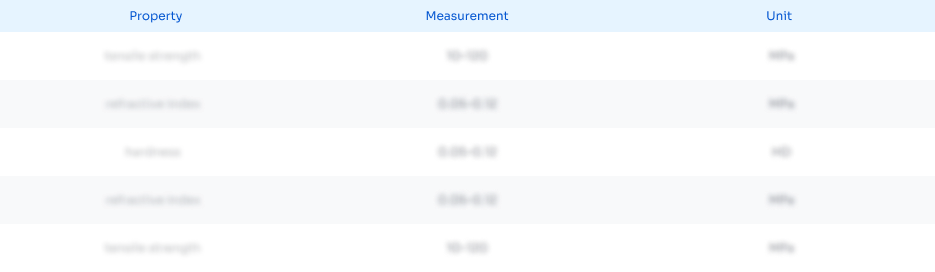
Abstract
Description
Claims
Application Information
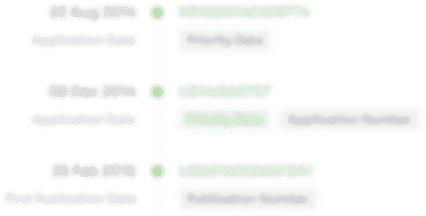
- R&D
- Intellectual Property
- Life Sciences
- Materials
- Tech Scout
- Unparalleled Data Quality
- Higher Quality Content
- 60% Fewer Hallucinations
Browse by: Latest US Patents, China's latest patents, Technical Efficacy Thesaurus, Application Domain, Technology Topic, Popular Technical Reports.
© 2025 PatSnap. All rights reserved.Legal|Privacy policy|Modern Slavery Act Transparency Statement|Sitemap|About US| Contact US: help@patsnap.com