Air pollution prediction method based on deep fusion of multi-source space-time big data
A technology for air pollution and prediction methods, applied in structured data retrieval, electrical digital data processing, digital data information retrieval, etc., can solve problems such as coarse ground resolution, large gaps, and influence estimation results, and achieve large space-time coverage. , a wide range of time and space, the effect of comprehensive influence factors
- Summary
- Abstract
- Description
- Claims
- Application Information
AI Technical Summary
Problems solved by technology
Method used
Image
Examples
Embodiment Construction
[0058] The present invention will be further described in detail below in conjunction with the accompanying drawings and specific embodiments.
[0059] The existing spatio-temporal estimation method of air pollutant concentration uses regression model estimation based on covariates, but the spatial resolution of meteorological covariates is limited, and there are a large number of missing values in remote sensing data, resulting in incomplete coverage of time and space; while capturing the source of pollutants The covariates of its spatio-temporal distribution are limited; many methods use black-box models for training models, lack of validity verification and uncertainty measurement, and there is no correction mechanism for prediction results. In this context, the present invention proposes an air pollution prediction method based on the deep fusion of multi-source spatio-temporal big data, which collects multi-source big data such as meteorological data, satellite remote se...
PUM
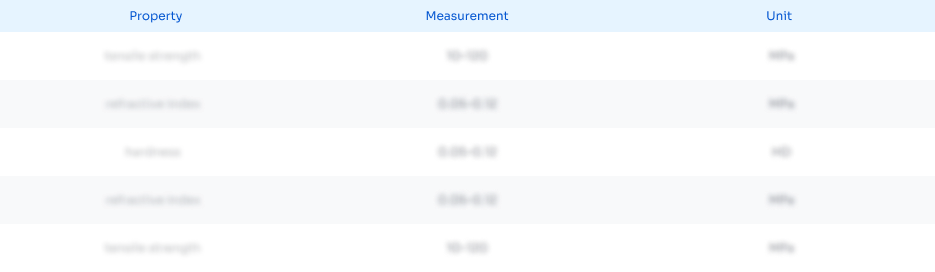
Abstract
Description
Claims
Application Information
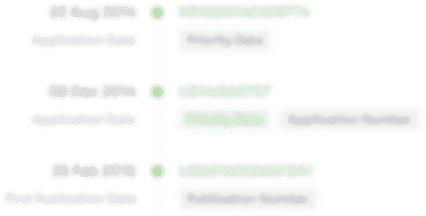
- R&D Engineer
- R&D Manager
- IP Professional
- Industry Leading Data Capabilities
- Powerful AI technology
- Patent DNA Extraction
Browse by: Latest US Patents, China's latest patents, Technical Efficacy Thesaurus, Application Domain, Technology Topic, Popular Technical Reports.
© 2024 PatSnap. All rights reserved.Legal|Privacy policy|Modern Slavery Act Transparency Statement|Sitemap|About US| Contact US: help@patsnap.com