Network traffic prediction method and device based on data reconstruction and hybrid prediction
A technology for network traffic and hybrid prediction, applied in the field of network communication, can solve problems such as being unsuitable for processing non-stationary nonlinear data, affecting the robustness of prediction models, increasing prediction complexity, etc., achieving high prediction accuracy and stability, avoiding Negative effects, effects that increase stability
- Summary
- Abstract
- Description
- Claims
- Application Information
AI Technical Summary
Problems solved by technology
Method used
Image
Examples
Embodiment 1
[0067] See figure 1 , figure 1 It is a schematic flowchart of a network traffic forecasting method based on data reconstruction and hybrid forecasting provided by an embodiment of the present invention, specifically including the following steps:
[0068] S1: Obtain network traffic value data.
[0069] In this embodiment, the flow value data of the set flow sequence X(t) at time t is expressed as X t .
[0070] S2: Reconstruct the network traffic value data to obtain the reconstructed network traffic value data.
[0071] See figure 2 , figure 2 It is a schematic diagram of a network traffic mixing prediction method based on data reconstruction provided by an embodiment of the present invention, wherein step S2 includes:
[0072] S21: Initialize network traffic value data to obtain multi-information network traffic value data.
[0073] Specifically, the time stamp corresponding to the network traffic value and the source information of the network traffic can be calcul...
Embodiment 2
[0138] On the basis of the first embodiment above, this embodiment provides a network traffic prediction device based on data reconstruction and hybrid prediction, please refer to Figure 6 , Figure 6It is a schematic structural diagram of a network traffic prediction device based on data reconstruction and hybrid prediction provided by an embodiment of the present invention, which includes:
[0139] Data acquisition module 1, used to acquire network traffic value data;
[0140] A reconstruction module 2, configured to reconstruct the network traffic value data to obtain reconstructed network traffic value data;
[0141] The decomposition module 3 is used to decompose the reconstructed network traffic value data by using the EMD method to obtain several network traffic value components;
[0142] Training module 4, for utilizing some network traffic value components to train the GRU-VTD neural network, obtain the trained GRU-VTD neural network;
[0143] The prediction modul...
Embodiment 3
[0146] The beneficial effects of the present invention will be further described through simulation experiments below.
[0147] 1. Simulation conditions:
[0148] The traffic data set used in this simulation experiment was provided by WIDE Internet’s Measurement and Analysis (MAWI) working group, extracting the average value of the data rate (in megabits per second (Mbps)) from 2014 to 2017, sampling The interval is 10 minutes, and the sampled network traffic data is used as the experimental data set. We use the data from January 2014 to November 2017 for training, and the rest of the data is used to test the trained model.
[0149] In this simulation experiment, the minimum acceptable flow rate in the missing point complementing step is 300 Mbps, the strength of outlier elimination is set to 6, and the number of IMF reservations in EMD is set to 3. Also, set the input flow length to one day (1440 minutes) and the predicted flow length to half a day (720 minutes), so set the...
PUM
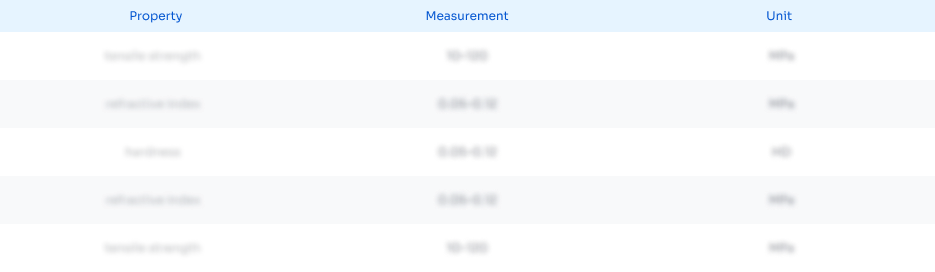
Abstract
Description
Claims
Application Information
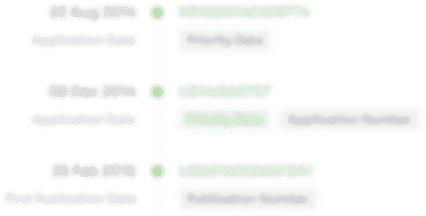
- R&D Engineer
- R&D Manager
- IP Professional
- Industry Leading Data Capabilities
- Powerful AI technology
- Patent DNA Extraction
Browse by: Latest US Patents, China's latest patents, Technical Efficacy Thesaurus, Application Domain, Technology Topic, Popular Technical Reports.
© 2024 PatSnap. All rights reserved.Legal|Privacy policy|Modern Slavery Act Transparency Statement|Sitemap|About US| Contact US: help@patsnap.com