Personalized recommendation method and system based on meta-path network representation learning
A network representation and recommendation method technology, applied in complex mathematical operations, instruments, data processing applications, etc., can solve problems such as inability to explain user-specific meanings and weak interpretability of matrix decomposition methods, and achieve improved accuracy and personalized recommendations Effects of performance, enhanced interpretability, and good application prospects
- Summary
- Abstract
- Description
- Claims
- Application Information
AI Technical Summary
Problems solved by technology
Method used
Image
Examples
Embodiment Construction
[0030] In order to make the purpose, technical solution and advantages of the present invention more clear and understandable, the present invention will be further described in detail below in conjunction with the accompanying drawings and technical solutions.
[0031] The ever-increasing heterogeneous data in the Internet can effectively improve the performance of the recommendation system. The main problem faced by the traditional matrix factorization model is how to integrate richer heterogeneous information data in the matrix factorization to improve the performance of the recommendation system. An embodiment of the present invention provides a personalized recommendation method based on meta-path network representation learning, see figure 1 As shown, it contains the following content:
[0032] S101. Constructing a heterogeneous information network by utilizing user social relations, user ratings on commodities, and category relations between commodities;
[0033] S102...
PUM
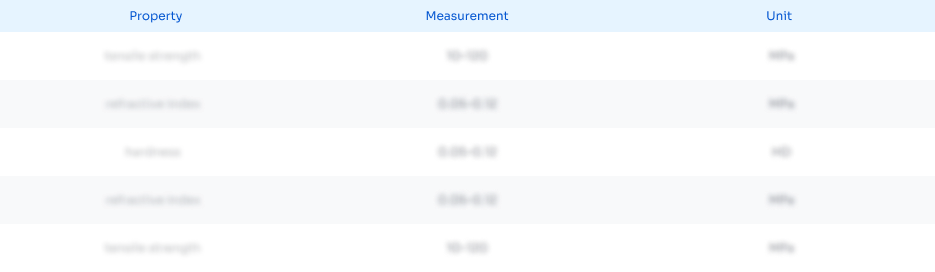
Abstract
Description
Claims
Application Information
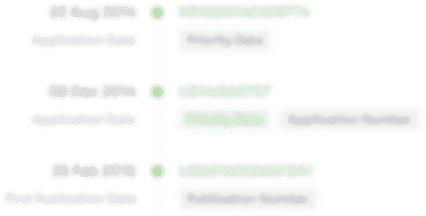
- R&D Engineer
- R&D Manager
- IP Professional
- Industry Leading Data Capabilities
- Powerful AI technology
- Patent DNA Extraction
Browse by: Latest US Patents, China's latest patents, Technical Efficacy Thesaurus, Application Domain, Technology Topic, Popular Technical Reports.
© 2024 PatSnap. All rights reserved.Legal|Privacy policy|Modern Slavery Act Transparency Statement|Sitemap|About US| Contact US: help@patsnap.com