Microbial marker for evaluating fecal microbiota transplantation curative effect of type 2 diabetes mellitus patients and application thereof
A technology of type 2 diabetes and fecal bacteria transplantation, applied in the field of microbial detection, to avoid excessive medical treatment
- Summary
- Abstract
- Description
- Claims
- Application Information
AI Technical Summary
Problems solved by technology
Method used
Image
Examples
Embodiment 1
[0044] Example 1 DNA extraction and sequencing
[0045] DNA extraction method: Genomic DNA was extracted from 0.25 g of fecal samples using the QIAamp PowerFecal Pro DNA kit according to the manufacturer's instructions. Processed samples are added to bead-beating tubes. Fast and thorough homogenization using mechanical and chemical methods. Once cells are differentiated, IRT is used to remove inhibitors. Total genomic DNA is captured on a silicon membrane in a spin column format. The DNA is then washed and eluted, ready for downstream applications.
[0046] Samples were subjected to 16S rRNA sequencing using an Illumina MiSeq.
Embodiment 2
[0047] Example 2 Identification of biomarkers
[0048] The identification and screening of biomarkers need to meet the following three conditions at the same time:
[0049] 1. The relative abundance of this bacterium is statistically different between healthy people and diabetic patients who are effective in fecal microbiota transplantation (before fecal microbiota transplantation); After fecal microbiota transplantation), there was no statistical difference.
[0050] 2. The relative abundance of this bacterium has no statistical difference between healthy people and diabetic patients who are ineffective in fecal microbiota transplantation (before fecal microbiota transplantation); After fecal microbiota transplantation), there was no statistical difference.
[0051] 3. The relative abundance of this bacterium is statistically different between diabetic patients who are effective in fecal microbiota transplantation (before fecal microbiota transplantation) and diabetic patie...
Embodiment 3
[0052] Example 3 Model Training
[0053] The constructed classifier is random forest. Using the relative abundance of the species as the risk value, the area under the curve (AUC) was estimated, and the larger the AUC, the higher the diagnostic ability. based on Rikenellaceae and Anaerotruncus Random forest classification performed at relatively rich levels yielded about 82.4% prediction accuracy with an AUC of about 0.83 ( Figure 7 ). The trained model can be based on the pre-FMT stool samples of patients Rikenellaceae Section and Anaerotruncus Abundance of genera was used to predict patient response to FMT treatment.
PUM
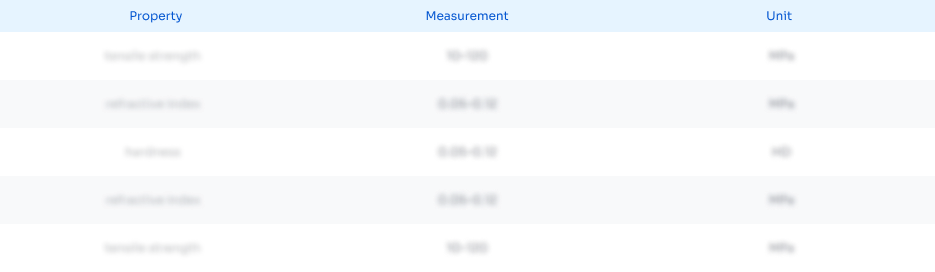
Abstract
Description
Claims
Application Information
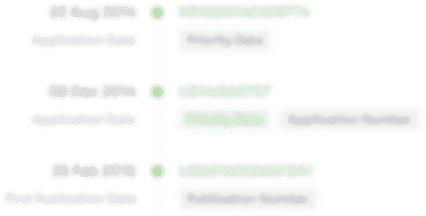
- R&D Engineer
- R&D Manager
- IP Professional
- Industry Leading Data Capabilities
- Powerful AI technology
- Patent DNA Extraction
Browse by: Latest US Patents, China's latest patents, Technical Efficacy Thesaurus, Application Domain, Technology Topic, Popular Technical Reports.
© 2024 PatSnap. All rights reserved.Legal|Privacy policy|Modern Slavery Act Transparency Statement|Sitemap|About US| Contact US: help@patsnap.com