User Scheduling and Simulated Beam Selection Optimization Method Based on Machine Learning
An analog beam and machine learning technology, applied in the field of wireless communication, can solve the problems of large scheduling scale, NP difficulty, and high computational complexity, and achieve the effects of high compatibility, improved classification accuracy, and high prediction accuracy.
- Summary
- Abstract
- Description
- Claims
- Application Information
AI Technical Summary
Problems solved by technology
Method used
Image
Examples
Embodiment 1
[0080] like figure 1 As shown, this embodiment provides a method for optimizing user scheduling and analog beam selection based on machine learning, and the method includes the following steps:
[0081] The user channel feature vector acquisition step: obtain each user channel feature vector from the user set to be processed in turn. The user channel feature vector includes the path loss, the 2L real-valued feature of the L complex path gain, and the L emission angles of the base station, where L is The number of channel channels between the base station and the user;
[0082] The analog beam matching step: sequentially input the channel feature vector of each user to the beam prediction model to determine the analog beam, specifically, using multiple beam classifiers to divide the downlink channel between the base station and the selected user into multiple different beam classes, Use the hyperplane to predict the category of the channel and select the best simulated beam fo...
Embodiment 2
[0142] This embodiment also provides a machine learning-based user scheduling and analog beam selection optimization system, including: a channel feature vector acquisition module, an analog beam matching module, and a channel scheduling module;
[0143] In this embodiment, the channel feature vector obtaining module is used to obtain the channel feature vector of each user;
[0144]In this embodiment, the analog beam matching module is used for analog beam matching, and the channel eigenvectors of each user are sequentially input to the beam prediction model to determine the analog beam, and multiple beam classifiers are used to divide the downlink channels between the base station and the selected user. For multiple different beam classes, use the hyperplane to predict the channel class and select the best analog beam for each user;
[0145] In this embodiment, the beam prediction model is trained by machine learning, and the training data includes a user channel feature vec...
Embodiment 3
[0187] This embodiment uses the optimization scheme of Embodiment 1 to compare the computational complexity. The comparison schemes specifically include a global optimal scheme (ES) based on exhaustive search, a local optimal scheme based on differential convex (D.c.) programming, and State-of-the-art low-complexity scheme based on greedy methods.
[0188] For the machine learning-based user scheduling and simulation beam selection optimization method in Embodiment 1:
[0189] The complexity of user classification is P(T uc KN RF N BS ), where T uc is the maximum number of iterations, T uc in express the base, Represents the set of support vectors for the svm classifier.
[0190] Therefore, the estimated complexity of this scheme is:
[0191]
[0192] Global optimal solution for exhaustive search:
[0193] Compute the feasible set of all user and beam pairs, the computational complexity is:
[0194]
[0195] in The general form is:
[0196] For locall...
PUM
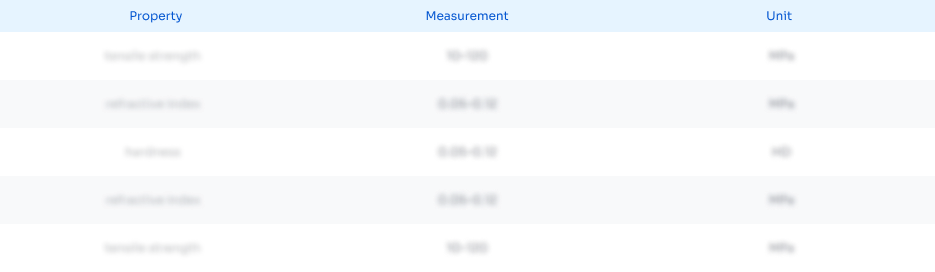
Abstract
Description
Claims
Application Information
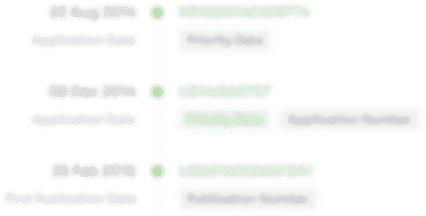
- R&D Engineer
- R&D Manager
- IP Professional
- Industry Leading Data Capabilities
- Powerful AI technology
- Patent DNA Extraction
Browse by: Latest US Patents, China's latest patents, Technical Efficacy Thesaurus, Application Domain, Technology Topic.
© 2024 PatSnap. All rights reserved.Legal|Privacy policy|Modern Slavery Act Transparency Statement|Sitemap