A Named Entity Recognition Method Based on Pre-trained Language Model
A named entity recognition and language model technology, applied in character and pattern recognition, neural learning methods, natural language data processing, etc., can solve problems such as poor effect, high labeling cost, poor model generalization ability, etc., and achieve fast , Improve the effect of accuracy, recall and F1 value
- Summary
- Abstract
- Description
- Claims
- Application Information
AI Technical Summary
Problems solved by technology
Method used
Image
Examples
Embodiment Construction
[0079] figure 2 is the overall flow chart of the present invention; such as figure 2 As shown, the present invention comprises the following steps:
[0080] Step 1: Build a named entity recognition system. Named entity recognition systems such as figure 1 As shown, it consists of a multi-model recognition module, a multi-level fusion module, a discrimination module, an entity label aligner, and an unlabeled database.
[0081] The unlabeled database D stores the text collection obtained from the Internet and other channels, including E texts, where E is a positive integer and 1≤E≤7000, and is connected to the multi-model recognition module and the discrimination module. The unlabeled database D is read by the multi-model recognition module and the discriminant module. D={D 1 , D 2 ,...,D e ,...,D E }, D e represents the e-th text in the untagged database; where N is a positive integer, D e represents text of length N (in characters, D e The length is N and the...
PUM
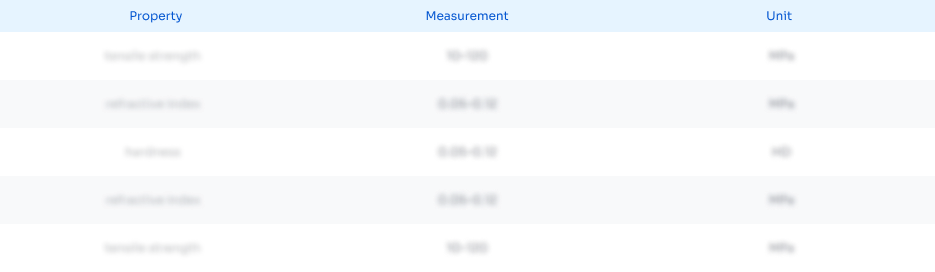
Abstract
Description
Claims
Application Information
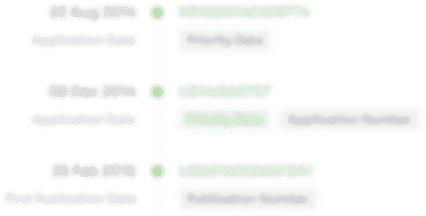
- R&D Engineer
- R&D Manager
- IP Professional
- Industry Leading Data Capabilities
- Powerful AI technology
- Patent DNA Extraction
Browse by: Latest US Patents, China's latest patents, Technical Efficacy Thesaurus, Application Domain, Technology Topic, Popular Technical Reports.
© 2024 PatSnap. All rights reserved.Legal|Privacy policy|Modern Slavery Act Transparency Statement|Sitemap|About US| Contact US: help@patsnap.com