Resource allocation method and system for multitask federated learning in 5G network
A technology of resource allocation and multi-tasking, applied in the field of machine learning, it can solve the problems that affect algorithm performance and convergence speed, affect the quality and correctness of federated learning parameter updates between users, and unreliable wireless channels, so as to ensure efficiency and accuracy. sexual effect
- Summary
- Abstract
- Description
- Claims
- Application Information
AI Technical Summary
Problems solved by technology
Method used
Image
Examples
Embodiment 1
[0052] In the multi-task-oriented learning process, there will be great differences in the execution time of different devices on different tasks, the amount of task input and output data, and task accuracy standards. It is necessary to comprehensively consider all aspects to ensure the total delay, energy or accuracy. The rate and other aspects have been optimized. This embodiment provides a user selection and resource allocation method for 5G multi-task federated learning.
[0053] The system environment is based on the 5G ultra-dense network scenario, and the specific architecture is as follows: figure 1 As shown, multiple user equipments are in the coverage area of the base station. Among them, the number of users is M, the user set is M=={1,2,3,...,M}, the number of task categories to be learned is J, and the set of federated learning task categories is J=={1,2 ,3,...,J}. All federated learning task categories that exist in the system need to be executed. All tasks e...
Embodiment 2
[0124] The purpose of this embodiment is to provide a resource allocation system for multi-task federated learning in a 5G network. The system includes:
[0125] The equipment data volume acquisition module is used to acquire the corresponding equipment operating parameters, local training data volume and transmission data volume for each equipment training task;
[0126] The device restriction acquisition module is used to determine the time delay and energy consumption required for each device to transmit each task in combination with the network environment parameters, the transmit power of each device and the device parameters;
[0127] The device screening module is used to screen the devices participating in this round of federated learning according to the time delay and energy consumption required for each device to transmit each task to the base station during each round of training tasks;
[0128] The resource allocation module is configured to allocate resources for...
Embodiment 3
[0130] The purpose of this embodiment is to provide an electronic device.
[0131] An electronic device, including a memory, a processor, and a computer program stored on the memory and operable on the processor. When the processor executes the program, it implements multitasking in the 5G network as described in Embodiment 1 A Resource Allocation Approach for Federated Learning.
PUM
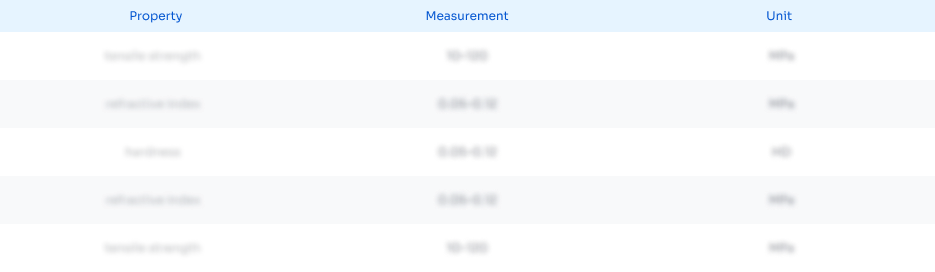
Abstract
Description
Claims
Application Information
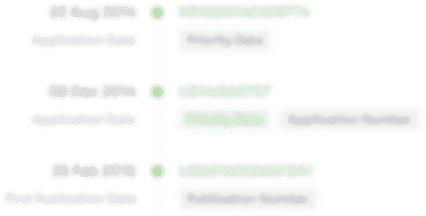
- R&D Engineer
- R&D Manager
- IP Professional
- Industry Leading Data Capabilities
- Powerful AI technology
- Patent DNA Extraction
Browse by: Latest US Patents, China's latest patents, Technical Efficacy Thesaurus, Application Domain, Technology Topic.
© 2024 PatSnap. All rights reserved.Legal|Privacy policy|Modern Slavery Act Transparency Statement|Sitemap